Preview – Most IBP cycles around the world are based on a 20-year-old process definition supported by 20-year-old planning concepts. This traditional IBP is not set up for a fast-changing world, where speed of decision making confers a competitive advantage. Intelligent automation will change this. In this series of blogs, I will discuss the evolution of traditional IBP towards a more intelligent IBP. Here, you can read the Rise of Autonomous , Unshackle the planners, Closing the Decisions Gaps and a new role for supply chain planners. In this blog: A Need for Decision Centricity.
THE TRADITIONAL FOCUS ON INFORMATION, NOT DECISIONS
In the evolution of IBP, there has been a lot of focus on the sequential planning process, meeting schedule, and planning capability. But it is good to remember that the early pioneers always designed S&OP, the predecessor of IBP, as an executive decision-making forum. Palmatier and Crum (2002), Coldrick, Ling, and Turner (2003) and Bob Stahl (2009) all assert that S&OP is first and foremost an executive decision-making process.
Decisions and decision practices are important, as decision effectiveness and financial results correlate at a 95% confidence level or higher. Which leaves Blenko and colleagues to conclude that “ultimately, a company’s value is just the sum of the decisions it makes and executes.“
According to a McKinsey survey, we spend on average 37 percent of our time making decisions, and more than half of this time is thought to be spent ineffectively. For middle management, ineffective decision-making reaches 68 percent.
One would expect a rigorous focus on high quality decision making in IBP. However, in my career as a consultant, supply chain manager or IBP manager, I have never encountered a formal executive decision approach to improve decision quality. Indeed, one study of 500 managers and executives found that only 2% regularly apply best practices when making decisions.
Incorporating Decision Quality
A decision quality framework is proposed in detail in the book Decision Quality. Below figure shows the framework with six proposed elements of decision quality. You can find detailed explanainations here.
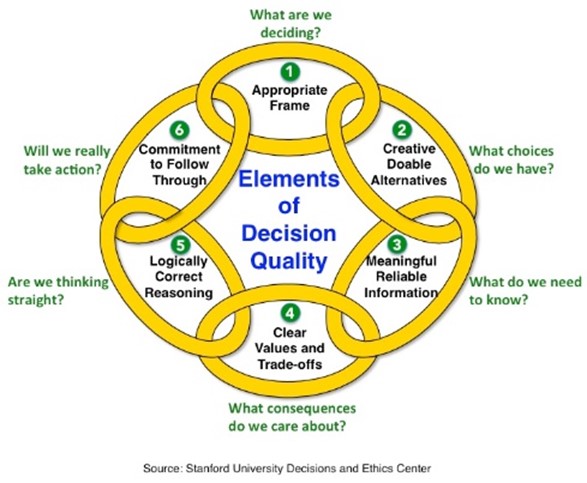
To measure decision quality, one can score all the six elements between 0% and 100%. The lowest score is the weakest link and will define the quality score of the decision. So if only one of the six elements scores 20%, the total decision quality is 20%.
To improve decision quality, organizations have to develop an understanding of decision models that include decision quality elements, support different decision types, explore if they are a cultural fit and provide value, and seek to integrate them in their operating model. Examples of decision models are the OODA loop for short term (S&OE) decisions, the Decision IQ framework for IBP decisions, the Decision Intelligence Navigator for strategic decisions, or the Casual Decison Diagram for unique decisions.
Besides increasing decision quality, benefits of good decision practices are reducing (non automated) decision time with 75%, reducing wasted analytics with 60% and improving employee engagement with 50%. Intelligent IBP will focus on the decision first and ought to include good decision practices.
Complementary Decision Strenghts
IBP decisions can be differentiated between machine centric S&OE decisions in the short-term horizon, and human-centric IBP decisions in the longer-term horizon (Regeer, van Hove, 2021).
We know from Moravec’s Paradox, that human and machine capabilities are complementary and from Kasparov’s law and that human-machine collaboration gives superior results. In the book Humachine it has been suggested that Human and machine qualities and strengths should be harnessed in a symbiotic relationship to outstrip performance achieved by either humans or machines alone.
Machine centric decisions will focus on automation and use machine strengths like computing power, frequency, speed, scalability, consistency, and endurance in decision making. The machine will free up time in the short term, so the human can spend more time on higher impact, longer-term IBP decisions.
Human centric IBP decisions can be augmented by a machine with supply-chain simulations, investment and risk modeling, product portfolio optimization, price setting, ware-gaming, megatrend analysis and even advice in negotiation strategies (van Hove, 2020). However, we must ensure to include human strengths the machine doesn’t possess, like creativity, empathy, innovation, contextual awareness, relationship management, meaning and purpose in decision making.
In our intelligent IBP decision process, we do not only include Decision Quality, but also human and machine decison making strengths.
Human Decision Weaknesses
We are wired to “satisfice” and settle for ‘good enough’ rather than the best decision. Psychologists and behavioral scientist have identified over 200 human biases. Many of them impact individual and group decision making. Based on their combined experience, decision quality experts Spetzler and colleagues (2016) identified five mega biases that are threats to good organizational decision making.
Narrow Framing: poor framing is the most common and value destructive source of decision failures, with narrow framing the most problematic frame trap. With a bias for action and quick results, we choose a frame that is too limited. As a result, we are set up to solve the wrong problem.
Comfort zone: the tendency to drag a problem into our comfort zone and solve the problem that we know how to solve, rather than the problem that needs to be solved. Again, leading to wrong or narrow framing.
Illusion of Decision Quality: the Decision Education Foundation (DEF), a not-for-profit that teaches decision skills, consistently finds that students overrate their decision quality. This overconfidence bias is also observed by executives who make multi-million-dollar decisions.
Agreement trap: this mega bias confuses agreement with a good decision. Without rigorously challenging the quality of a decision, we might end up with a consensus as defined by Margaret Thatcher; “The process of abandoning all beliefs, principles, values and policies in search of something in which no one believes, but to which no one objects”.
Advocacy/approval myth: the mistaken believe that a quality decision can be reached by relying on powerful advocacy and intense questioning. If a recommendation is presented by advocates, quality is either there in the recommendation, or it isn’t. Questioning can only improve the decision if it is focused on the six decision quality elements.
Especially the last two mega biases seem to be incorporated in IBP by design. There is a high focus on reaching consensus (agreement) in meetings where the IBP teams advocate their recommendations and executives skillfully drill down in the detail through questioning, often without an explicit focus on decision quality.
An intelligent IBP decision process does not only exploits human and machine decision strenght, it also ought to prevent human weaknesses in decision making.
Decision Intelligence Technology
There are simple checklists that can speed up and make better IBP decisions and improve decision quality. However, to fully exploit human and machine strenghts and prevent human weaknesses, and learn from decisions, we want to look to technology for some help as well
I previously highlighted that we need a third Wave of integrated supply chain planning software (van Hove, 2019) that goes beyond Wave 1, Enterprise Resource Planning (ERP, system of record for master & transactional data) and Wave 2, Advanced Planning Systems (APS, system of record for planning data & assumptions). This new wave is required to support faster and higher quality IBP decision making as well.
Although both ERP and APS systems support forecasters and planners to impact decision quality, they are focused on the inputs to a decision, like data, analysis, insight, forecast, plans or scenario’s, not on the decision itself. Systems of Intelligence will become the sytem of record for automation, augmentation, human cognition, decisions, actions and learning. Decision Intelligence Technology is a Wave 3 type of technology.
Decision Intelligence technology treats the decision-making process, the decision itself, the decision maker, decision value, decision impact and all the decision context as data points that can be digitized, captured, shared, visualized, analyzed, audited, and learned from. Once the decision is treated as a data point, this technology can apply machine learning to estimate the likelihood of decision success and impact, and to continuously improve decisions, hence improving its quality.
These types of capabilities can only be provided by technologies that are built around the decision. The average forecaster or planner will understand that the wave-one or wave-two technologies they are working with can’t provide these decision capabilities. Luckily, the first providers of decision intelligence technology have arrived, and we’ll see an acceleration of these types of technologies in the coming years.
Conclusion
One of the evolutions in Intelligent IBP, is the a focus on and integration of decision quality in the IBP cycle. Improved decision quality can be achieved through an integration of best practice decision processes and checklists, supported by decision intelligence technology that digitizes the decision process to create a system of record for decisions. Using this approach, intelligent IBP will make faster, higher quality decisions, whilst reducing analytics waste, increasing employee engagement and continuously learn from decisions.
Hi Niels – Excellent Blog and expertly written as always. I am quite passionate about this subject and fully agree that the next wave of IBP has to be about realising the full potential of the process (as originally intended) through effective decision making. Understanding how to position for decision quality (DQ) is critical supported as you say by intelligent automation or augmentation depending on the type of decision. At the executive level in particular I believe there are other elements in play to achieve the shift in collective mindset required to ensure there is full trust to make the decisions that carry risk, but also great rewards. With practice executive teams can get better at it and will be motivated once they realise that it can deliver a competitive advantage. However executives need to be educated such that they have a deep understanding of how to deploy the core IBP principles to build the required trust. When combined with DQ and intelligent augmentation they are then ready to maximise the value from their IBP process.
Thanks for your feedback Jerry and I agree. For the big decisions there is much more to explore. Not just decision quality and the prevention of bias, but a collective courage to take calculated risk. We’re well into the area of psychology there. Under continuous scrutiny of the market it is so easy to choose the easy or less risk full option and miss the opportunity for big rewards. Let’s keep the conversation going.
Ultimately it is about creating what I like to call the ‘Decision Imperative’. How can it become so obvious that a decision needs to be made, such that the risk of not making a decision is considered greater than that of making a decision. I believe the clues on how to achieve this can be found by investigating the circumstances when decisions are triggered by what are often emotional responses to the here and now. For example: “Quarter results are not where we need to be so we need to cut costs – let’s ban travel and put a hiring freeze in place”. Such decisions are made in an instant and while they may not be the correct decisions, there is a learning on how to engage a similar need to make a decision about a potential issue that is out into the future. If can engage that emotion supported by DQ and intelligent augmentation then think we are on the right path.