The IBP paradigm has hardly changed for 30 years, the decision needle has not moved enough.
We’ve entered an AI era where 74% of work can be augmented or automated and 26% eliminated. Planning will not be excluded from this evolution.
However, only 3% of companies apply automated execution and 7% autonomous end to end planning. 2% of managers apply best practice decision methods. Hardly a recipe for improving planning & decision making.
The idea of Intelligent IBP is to close these planning, decision & execution gaps and grasp the opportunities that comes with it. In this manifesto I will introduce Intelligent IBP principles. Many which I’ve highlighted over the years in blogs and articles.
Introducing Intelligent IBP
Let’s start with a definition.
Intelligent IBP effectively combines human and machine strengths with good decision practices to consistently make timely, high-quality, horizon agnostic decisions.
This definition should become clear once you go through the principles. These principles are a first guide around the WHAT, not necessarily the HOW of Intelligent IBP. It will certainly not address underlying planning concepts like demand planning, MPS, inventory management, production scheduling, MRP, etc etc.
It will describe an IBP vision on where we could go when human and machine work together as one. A new planning paradigm. Adaptions to the realities of todays world, using the possibilities of todays technology. These are ideas of enhancement, evolution, not replacement.
In the end, IBP is a philosophy, or belief system that strives for transparency, near perfect integration and alignment, both horizontal (between functions, BUs, even companies) and vertical (between strategy, planning and execution). This is impossible, however, we can achieve a critical mass of integration and alignment that creates benefits. And we sometimes already do.
I think it is time to update the IBP belief sytem to be more reflective of current times, technology & new ways of working, and create a modern IBP vision.
New IBP principles can result in an evolution of IBP that leads to the creation of new supporting operating models (Organizational design, processes, roles & responsbilities, incentives, measurement framework, mindset & behaviours, technology enablers) that strive to get the most out of machine strenghts and human strengths, whilst minimizing human weaknesses and increase human engagement.
These new operating models can create significant benefits. We will decide and respond faster and more accurate in our supply chain and make higher quality operational and strategic decisions.
Supporting the planner
My main interest however, is to give planners a more interesting and engaging job and career, free up 40% of their time to work on interesting stuff, rather than tinkering for 50% of their time with data, replan, replan, replan and make IBP decks. See below slide from one of my webinars.

I was a planner once. The role of the planner has not evolved enough. We have an opportunity to change that. The job of the planner in the above slide is more enticing than what I still see today. I believe that soon enough it will become a must to provide better roles to young graduates for them to be interested in working in the supply chain, or for your company.
An updated belief system and new operating models won’t solve all of the issues that holds IBP or any change effort back. Vision, strategy (lack of it), cultural, leadership, behavioral, skill and mindset challenges won’t magically disappear. They might even get more challenging when we put the machine more and more in the mix of new operating models.
However, standing still and sticking to old IBP dogma’s, not innovate, come up with new ideas and envision a better supply chain planning future is not an option for me.
Intelligent IBP in the making
As early as 2016, I wrote about autonomous IBP, comparing IBP to an autonomous car. Then, as a response to the ‘touchless planning’ hype, I published an article in 2019 about what is really required for ‘autonomous planning’.
When my colleague Hein Regeer and I in 2021 envisioned a new type of IBP, where decisions are segmented between humans and machines, with increased levels of planning and decision automation and augmentation, I was pleased with the feedback from supply chain professor Nada Sanders:
You offer an excellent path toward reinvention of traditional IBP that companies should follow.
Nada is co-author of the book HUMACHINE besides plenty of other books. Alain Perrot, a 30-year IBP veteran with over 200 implementations on all continents in all industries, and developer of the Value Race, congratulated me with changing the paradigm, but also challenged me to describe a full planning symbiosis between human and machine. I had a go at that with this blog and Alain’s feedback after reading it was:
Truly a great article , Niels, congrats. Like any pioneering thinking, you need to find a customer to make this breakthrough thinking a reality.
When I thought about what I could call this evolution of IBP, I simply landed on Intelligent IBP, as I believe both machine and human intelligence have to be exploited more. And artificial intelligence (AI), intelligent agents and a more intelligent approach to human decision making will all be part of the solution. In March 2022, I wrote the first of five Intelligent IBP blogs.
However, what we call this evolution of IBP is not important. Progress, a new enticing planning vision, and a better future for planners are.
Enter Decision Intelligence
Working for Aera Technology, applying intelligent automation to decision making, helped shape these early ideas further since I joined the company end 2019. The launch of a Decision Intelligence (DI) definition by Gartner later in 2022 further cemented my believe that this discipline will become mainstream.
Aera defines decision intelligence as “the digitization, augmentation, and automation of decisions.” In that order, because we first have to be able to orchestrate and digitize the decision process, including all data, plans, parameters, and decision policies that feed into it, before we can augment or automate.
Google’s Cassy Korzakov already had her DI definition in this blog from 2019, and Lorien Pratt published the book Link about decision intelligence in that same year. These two ladies are real Decision Intelligence trailblazers, both have roots in AI/ML. A lot of good decision practices go back to the pioneering paper; Decision Analysis: Applied Decision Theory from Ron Howard in 1966.
Cassy defines Decision Intelligence as “the discipline of turning information into better actions at any scale”, highlighting that the days of information, insights and BI are over, or at least not enough anymore. We need decisions and actions.
She further defines a decision as ’any selection between options by any entity.’ Cassy highlights that there need to be multiple options to choose from and she opens up the door that a machine might as well make that decision.
We all stand on the shoulders of giants. What I learned as decision intelligence practitioner and Foresight editor, is that you can look at it from many angles as there is such a wide range of decision types and circumstances out there. And there a plenty of different sciences and management practices to support or improve it. So, there are many possible approaches.
This manifesto simply applies it to the range of decisions covered by supply chain planning & IBP using a synthesis of the HUMACHINE idea, decision intelligence, good decision practices, effective mindsets combined with my own IBP experience and vision.
Principles of Intelligent IBP
Core Principle 1 : Intelligent IBP proposes ideas of enhancement and evolution, not replacement of traditional IBP.
Intelligent agent: In artificial intelligence, an intelligent agent is an agent acting in an intelligent manner; It perceives its environment, takes actions autonomously in order to achieve goals, and may improve its performance with learning or acquiring knowledge (Wikipedia)
Core Principle 2: Intelligent IBP uses intelligent agents to advance planning and decision making.
Core Principle 3: Intelligent IBP seeks a full symbiosis between human and machine to make decisions; however, the human will always be leading.
A Decision Centric Approach
Although IBP is an executive decision-making forum, historically the focus has been on information, process & planning. Not so much on decision making, decision quality and good decision practices. Unfortunately, only 2% of managers apply best practice decision practices. Additional benefits of good decision practices are reducing (non automated) decision time with 75%, reducing wasted analytics with 60% and improving employee engagement with 50%. Intelligent IBP will focus on the decision first.
Principle 1: Intelligent IBP takes a decision centric approach and has a thorough understanding of decisions to be made, decision quality and good decision practices.
Decision Cycles
In a 2020 Foresight article, I highlight the different horizons in common business planning cycles and how they are related. Based on six planning & decision drivers (frequency, data generation, granularity, impact, system complexity, human centricity), I then extract the relative efficacy of automation (in both process and decisions) vs. augmentation across the business planning horizons. I conclude that the longer the planning horizon, the more human centric decisions will be.
Figure 1: Business planning cycles
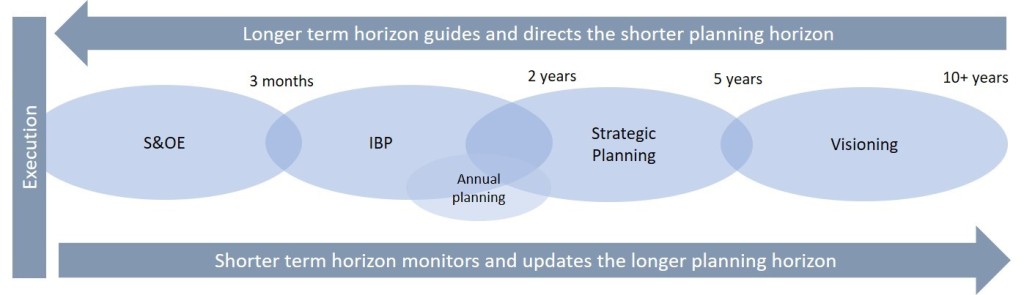
Figure 2: Six automation and augmentation drivers and their relative impact by planning horizon
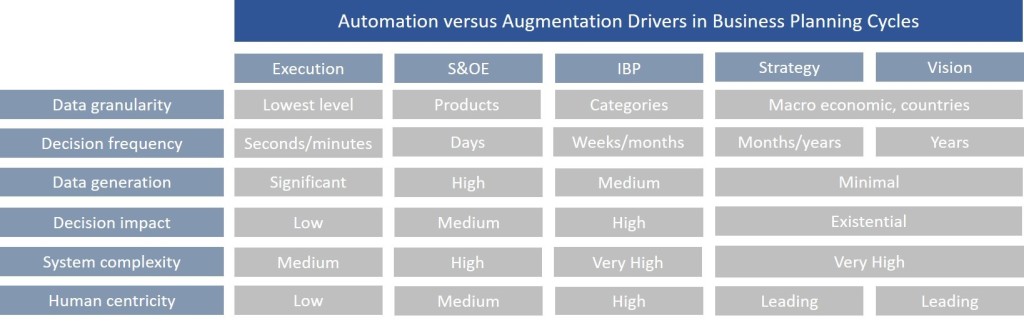
Figure 3: Relative preference for automation & augmentation by business planning horizon

Principle 2: Intelligent IBP is horizon agnostic and can be applied to every business planning & decision cycle.
Decision Types
In a 2021 commentary on an article about the HUMACHINE, I segmented decisions in operational, planning, strategic and cultural decisions. I would now split planning into basic planning decisions (change a price to close a projected value gap) and complex planning decisions (align internal functions, supplier, and customer around a new product introduction, relationship complexity that a machine can’t solve).
In my presentation at the 2021 International Symposium on Forecasting (ISF), I then combined decision types with augmentation and automation efficacy as per figure 4 (which I would slightly change now: I would differentiate between augmented analytics and decision augmentation).
Figure 4: Slide from the ISF 2021

There are other ways to segment decision types, as per suggested McKinsey decision types here. Smaller decisions that are ripe for automation and too much for the human to handle, are also called ‘micro decisions’. The point is that to describe a full symbiosis between human-machine across horizons and decision types, we need to map all decision types and decide what’s part of the intelligent IBP decision scope. Even when there is no significant planning involved for a decisions, other types of augmentation (machine support) are still possible to support the human.
Principle 3: Intelligent IBP includes every decision type across business planning cycles.
Decision Segmentation
To get the most out of human and machine, and get a feel for their interaction, it is key to segment IBP decisions in machine centric IBP decisions: guided by the human and Human centric IBP decisions: augmented by the machine. This is what my colleague Hein Regeer and I proposed in our 2021 Foresight article (See figure 5). Our suggested segmentation can be further refined with segmentations that call out Human in the Loop (HITL), Human in the Loop for Exceptions (HITLFE), Human on the Loop (HOTL) and Human Out of the Loop (HOTL), (James Taylor, 2021, HBR).
A clear decision segmentation and a company’s choice on what decisions segment(s) to excel, will drive the strategy and roadmap for planning & decisions automation & augmentation and the supporting operating model.
Principle 4: Intelligent IBP segments between machine-based decisions, and human-based decisions.
Figure 5: IBP decision type segmentation by human and machine

Decision automation versus augmentation
What decisions can/should be automated?
Not everything can or should be automated. But where we can, we have to consider it. Data gathering & cleansing, descriptive analytics, dashboards, reporting, operational and basic planning decisions, can be automated where possible (mostly in the S&OE horizon, but also beyond). Master data maintenance can be highly automated. Planning parameters (like a lead time, safety stock, etc) can be maintained continuous and become probabilistic in nature. Short and mid term demand & supply balancing decisions, can be automated, including volume and value gap closure.
A Gartner report suggests that by 2026, over 65% of short-term decisions within supply chain planning will be automated or autonomous. Planning functions with a majority of decisions being automated or autonomous are sales and operations execution (S&OE) (57%), order fulfillment/available to promise (56%), production planning (53%) and production scheduling (53%).
By digitizing planning and recurring decisions, its inputs, trade-offs and goals, many decisions require less collaboration. Pre-defined, agreed, maybe cross functional negotiated decision boundaries and trade-offs can be provide to the machine (intelligent agents). The machine will take these negotiated boundaries into account when augementing or automating decisions. In these video’s (1-2 min), I explain the automation of routine collaborative decisions, executional decisions, planning parameter decisions, ESG decisions and segmented decisions
Principle 5: Intelligent IBP automates repetitive planning tasks, basic planning and transactional decisions.
Principle 6: The human maintains goals and boundaries on where and how the machine should automate.
What decisions should be augmented?
Where automation is not possible or the preferred option (too high value or impact, contextual or relationship complexity), the human leads and the machine helps the human by automatically providing multiple options to choose from. I see two steps in augmentation:
In these cases, the machine can still augment the human with analytics, providing options, what if’s, long term trend analysis, scenario’s, simulations, war games to choose from. It is less likely to give ‘advice’. Still, providing the different options to choose from before deciding, creates insights and improves decision quality.
1. Augmented analytics runs (automated) advanced analytics to provide unique insights or different possibilities to a planner/user. For example a monthly triggered risk analysis (set of simulations) from your e2e supply chain. No suggestion or decision will be made, just insights.
Human centricity remains paramount around cultural decisions & visioning. It is the only basis for defining purpose, values, and behaviors, but also sustainability, corporate responsibility, diversity, and inclusion, elements which are beyond the reach of the machine. Augmented analytics here is possible, augmented decision making not so much.
I see more strategic decisions (close a plant or DC, enter a new product category, change in strategic sources) more in this category as well (which is different than what I show in figure 4).
Another examples of augmented analytics is where a user can type an analytics question in to a planning system, where the planning system use ChatGPT type of technology (large language models), to provide an answer. These machine answers create insights, not decisions.
2. Augmented decision making. This is where the machine gives an ‘opinion’, advice or recommendation to the human about the best choice to make between different options but leaves it up to the human to agree or not.
These will be operational and basic planning decisions that otherwise could be automated, but go beyond a certain threshold. Hence the human needs to be in the loop to make the final decisions, but the machine will give the ‘advice’ around what’s best in the circumstances (which it might have learned over time).
Add to this more complex planning decisions (For example with relationship complexity, NPD or price decisions with suppliers and customers). The machine can automate a price change for an online retailer easily. However, for a manufacturer a price or promotional change might first need to be aligned with the customer. Hence the machine can give this advice, but the human need to stay in the loop for the final decision.
All augmentation itself can be automated through a time, variance, probability trigger, or if not, by an ad-hoc trigger by the human. These are rough guidelines. In the end, every business has to take a decision centric approach and decide what can be automated and what should be augmented.
Principle 7: Intelligent IBP augments the human by providing multiple options for decisions that are not automated.
Principle 8: The human provides the boundaries on where, when, and how the machine should augment.
Recurring Decisions
Many planning & IBP decisions are repetitive. Maybe not frequent, but they have been made before. If it is not in your business or industry, it will be in another industry or business. Most business decisions are not as unique as we would like to think. This means we can digitize and orchestrate many of them and work our way back what is needed to make this decision in the best way possible, including decision quality & bias reduction.
Principle 9: Intelligent IBP maintains a digitized library of decisions, how they are made, what the common options are, what data inputs and analytics are required and who decides.
Documenting recurring decisions like this creates alignment and transparency in the decision process, increases decision speed and reduces analytics waste for human centric decisions. It also increases employee engagement as the how and what of decisions are clarified, reducing meeting time, unnecessary collaboration, and politics to favor certain options.
To define and digitize the many possible decisions in a transparent and consistent way across the enterprise, we want to have a common structure or language to define decisions, like for example the Decision Model and Notification (DMN).
Principle 10: Intelligent IBP uses a common, structured notation/language to define decision inputs, steps and the decision itself.
Below picture shows an overview of demand & supply balancing options in a global beverage business. It’s over a decade old. Many decisions will be familiar, even across industries. The options to choose from will largely be known, so are the data, plans & insights required. An example on how to map a decision tree based on functional goals and business issues is given in this Decision Back whitepaper.
Intelligent IBP will have the objectives and decisions trees documented and highlighted where there is automation and augmentation potential. Per decision, the decisions inputs, steps and decision making are then documented and digitized with a common, structured notation.

Unique Decisions
If we encounter complex strategic or unique high impact decisions, a Causal Decision Diagram (CDD) can be used, as defined by Lorien Pratt. A CDD is worthwhile if the time and costs to develop a CDD and simulate decisions and outcomes is worth the benefit of the decision impact. It is wise spending time and money on a one off billion $ decision, or a complex, high impact recurring decision. More basic trade-off decisions don’t need a CDD and can use a simpler type of decision design. Once a team works through the CDD, the decision is defined and can be digitised in a decision library.
For new decisions, The Decision Intelligence
Navigator from Dr Roger Moser guides a user through decision context, appropriate framing, and intelligence access, hereby structurally addressing decision-quality elements and helping a decision maker how to best set up a decision and choose a decision approach.
Principle 11: Intelligent IBP uses a common, structured approach to decide the best approach for making new decisions.
Human & machine strengths
Moravec’s paradox describes that human’s and machine have complementary strengths. Intelligent IBP will need to exploit both strength in human & machine segmented decisions.
Human centric decisions can include human qualities like creativity, empathy, innovation, contextual awareness, relationship management, meaning and purpose. Creativity (brainstorming) and innovation are important to seek multiple, or new options/angles to solve issues. Idea generation can be supported by generative AI. It’s easy enough these days to ask ChatGPT for some ideas or ask DALL E2 for some visuals in sketch or digital art from. Having multiple options is an important element of decision quality. Empathy and relationship context are important for human change and implementation/execution aspects of decisions.
Machine planning & decisions will focus on machine strengths like computing power, frequency, speed, scalability, consistency, and endurance.
Principle 12: Intelligent IBP exploits machine strengths in automation and augmentation
Principle 13: Intelligent IBP seeks to exploit human strength in human centric decision making.
Human-Machine collaboration & trust
Kasparov’s law dictates that a good process between and average human and machine is superior to great machines and great humans with an average process between them. Hence, human-machine collaboration becomes critical.
It should be easy for the human to provide goals and automation and decision policies to the machine. It should be easy for the human to receive and understand advice from the machine in order to make and execute a decision. We need a good UI/UX for planners to type in some commands or questions, Voice technology is a must as well, beyond that eye control as a cursur and maybe one day Elon Musk’s Neuralink Brain chip.
Principle 14: Intelligent IBP requires an effective, near frictionless human-machine interface to communicate
A good human-machine process builds trust and enhances collaboration, but to maintain that trust, the human also needs to understand to some extent what the machine does (although, how many supply chain professionals can really explain how exponential smoothing works, or Kings formula for safety stock?).
Principle 15: Intelligent IBP provides explainability for automated and augmented decisions.
Good Decision Processes
For human centric and augmented decisions, emphasis will be put in negating the over 200 human biases in decision making. Good decision processes can minimize human biases and should be used to get the most out of human strengths. Althought speed of high impact decisions (which should be the only decisions in an exec IBP meeting) is still relevant, the reduction/prevention of bias for high impact decisions is critical. Bias reducing decision practices are a major gap in traditional IBP.
However, there are other benefits. Organizations that follow best practice decision checklists make decisions twice as fast in half as many meetings, find innovative solutions 75% more often and generate 20% better performance. The UK Institute for Employment found that decision-making effectiveness impacts 50% of overall employee engagement, directly affecting productivity and retention. There can be up to 50% reduction in wasted analytics.
Decision IQ has been proposed as the combination of the decision process + decision execution. It focuses on a mid range of decision making, between the handful of major strategic decisions, and the dozens of daily trivial (micro) decisions. A 2023 study of 160 companies found only 15% of companies excell at both. Process and execution are both important, but improving the decision Process offers the greatest opportunity for improved business outcomes. Below figure shows the suggested process from the study.

Principle 16: Intelligent IBP uses decision processes that speed up decision making and minimize human bias and analytics waste
Decision Quality
Although IBP is an executive decision-making forum, there has been limited focus on decision quality. Decision quality will be included in the design for automated decisions making. There need to be controls for machine ethics and bias in automation and augmentation. Although machine bias has a trade-off against variance, so that’s more optimizing both, rather than only minimizing bias.
For human centric decisions, it will be part of the orchestration process for high impact decisions. Decision quality will become a performance metric in IBP. Similar to Cassie Korzakoff, authors of the book Decisions Quality, there need to be multiple options (they argue these need to be significantly different) to choose from, otherwise a choice is not a decision.
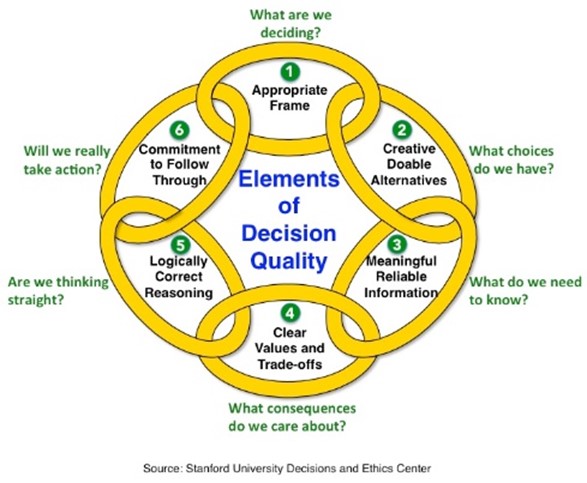
Principle 17: Intelligent IBP seeks to integrate decision quality for every decision type.
Principle 18: Intelligent IBP integrates decision quality in the design of automated decisions.
Principle 19: Intelligent IBP seeks to optimize machine bias in decision making.
Principle 20: Intelligent IBP measures decision quality and decision impact for every decision type
IBP decisions and its impacts will need to be digitally recorded, be transparent, accessible for al IBP stakeholders, and be able to learn from decisions over time (by applying machine learning)
Principle 21: Intelligent IBP aims to digitize every decision type (even 100% human decisions) and decisions made
Principle 22: Intelligent IBP learns from decisions made and has the ability to feed these learnings back to the human.
The IBP organizational design
Digitization and automation will create new types of organizational designs, including for the supply chain. There will certainly be changes in the planning and IBP organizational design. The IBP team will likely remain, but I foresee the additional changes in roles and responsibilities.
Decision squad: I foresee the creation of a decision squad to tackle urgent, high impact decision, that otherwise simply can’t be solved timely and with enough quality in the IBP cycle. The decision library will define what decisions this squad will be assigned to and what roles will be in the squad.
IBP product owners: I foresee IBP product owners that are accountable for one or more intelligent IBP agents that support with (autonomous and augmented) decision making. They are accountable for facilitating and maintaining the boundaries of decisions making. This McKinsey article describes an example for a future digital supply chain organization. The ‘Digital Twin Engineer’ is similar as an IBP product owner.
Other Roles & responsibilities: the role of the planner will change as I describe in this blog. We will see the birth of the decision role and the automation role, and we see how the planning & information role will become less transactional and more strategic. Field research from the Kuhne Logistics University, shows interesting insights on newly expected planning roles, like supply chain architect, cognitive analyst and data manager. Some of the ideas around these merging roles are clearly overlapping.
The end result however, is that there will be less planning roles, working with the machine covering more planning scope, supported by new emerging roles to improve automated and augmented planning.
Think about this anecdotal example of a very large CPG with a portfolio of 25,000 SKUs with 800 planners for a certain geography, versus an mostly digitized online retailer with 5 million SKUs, 15 planners and 150 analysts. Sure, they have different supply chains to manage and to plan, but you can guess what the long-term trajectory will be for the planning structure in the CPG.
Principle 23: Intelligent IBP minimizes dull repetitive planning tasks and makes planning roles more strategic and more value adding.
Principle 24: Intelligent IBP requires new and changing roles for a planner.
As the machine takes over more and more tasks from the planner, we will see examples of the machine – a specific intelligent agent that solves a planning problem – report into a human.
Principle 25: Intelligent IBP requires an organizational design, where the machine can play a specific planning role and can report in to a human planner.
Planning incentives: IBP incentives (or rewards & recognitions) will shift from policing process compliance like in traditional IBP, to provide strategic business scenarios, find incremental levels of planning & decisions automation and augmentation. Measure and improve decision quality.
Principle 26: Intelligent IBP requires new incentives for planners around decision automation, augmentation, speed, quality and learning capability.
The IBP cycle
Data and Analytics: A planner spends roughly 50% of the time on data gathering, cleansing, tinkering, before even coming to a conclusion, a recommendation, or a decision. This is a waste of time and human capital and not an engaging role for a planner. We should aim to highly automate these tasks. The following four principles were suggested in this article I wrote with my colleague Hein Regeer.
Principle 27: Intelligent IBP will automatically gather and cleanse relevant internal and external data and distribute these across the business in one common data layer, in support of planning & decision making.
Principle 28: Intelligent IBP will automatically provide descriptive and diagnostic analytics and make these available at any time throughout the business.
Planning: The times of siloed, sequential, time segmented planning are over. To speed up decision making we need to find ways to connect longer term planning to shorter term planning & execution. I sometimes question the need for an S&OE horizon and S&OE cycle. A machine doesn’t really care in what horizon a decision needs to be made, or at what product level. These horizons and hierarchies are made up by humans to make planning live easier and manageable. We might need some horizons where we want more stability (frozen zones etc) to control potential erratic automation behavior.
Principle 29: Intelligent IBP plans continuously and requires human input only upon matters that exceed certain impact, probabilities, or complexity thresholds
Principle 30: Intelligent IBP will identify variations and gaps to plan and automatically action these up to a defined threshold. Above that threshold it provides recommendations to be decided upon by humans.
Principle 31: Intelligent IBP plans concurrent and horizon agnostic, rather than cascaded or time segmented.
For a long time, it has been suggested to use a range of planning outcomes to make IBP decisions rather than a point plan, the one number illusion! Probabilistic planning now provides the opportunity to display this range as a distribution function. This automatically provides decision makers with scenarios to think opportunistic (demand and supply operate at >95%) and at the same time prepare for pessimistic scenarios (demand and supply operate at <5%). This improves decision quality and business readiness.
Principle 32: Intelligent IBP uses probabilistic planning to provide an input and output range for decision makers.
Monthly versus Ad-hoc decisions: The focus on monthly decisions is a shortcoming in traditional IBP. It’s simply not always a decision cycle a business operates at, even for strategic decisions. Ad-hoc, high impact decisions can be facilitated outside the IBP cycle but will stay aligned. I described this in my 2023 Foresight article using the diagram below.
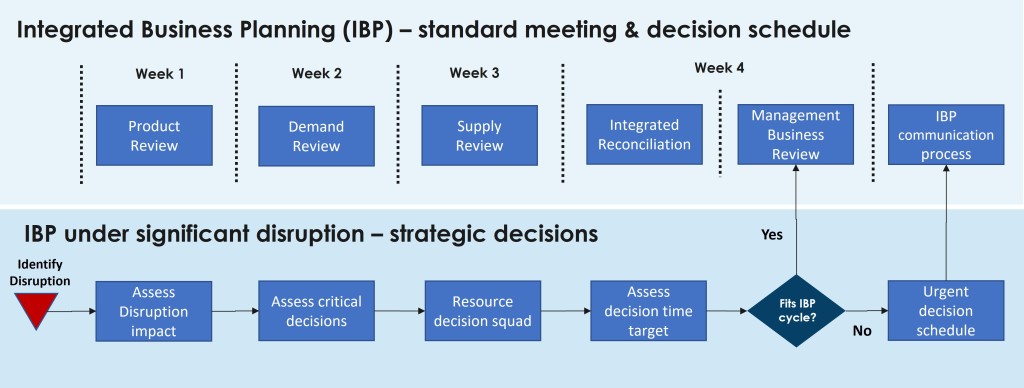
Principle 33: Intelligent IBP can make decisions at any time required but stays aligned with a monthly cycle.
IBP meetings: The frequency and duration of IBP meetings will be more driven by decision requirements, rather than fixed process requirements.The traditional, sequential, planning cycle (PR, DR, SR, IR, MBR) will change for something more continuous. I still see value for humans to come together in a monthly cycle and apply human strengths (and minimize bias) to business problems and decisions. I foresee that the demand & supply review will be more integrated in normal day to day operations, rather than a monthly meeting. The product review and the IBP meeting, due to the more strategic character, might maintain their periodic monthly character, but will have to change in scope and approach.
Principle 34: Intelligent IBP meetings are driven by decision requirements first and only secondary by process or calendar requirements.
Strategy integration: ‘The ultimate goal of IBP is the generation of a plan to support an organization’s efforts to deploy and execute its strategy’ (see my article on S&OP and strategy). A lack of strategy integration or strategic guidance, is also one of the main gaps in IBP. Often companies don’t even have a strategy plan, the strategic initiatives are not in the budget, most managers don’t know the strategy and even less employees understand it. One wonders if a company should even start an IBP journey without some of these strategic prerequisites in place. Intelligent IBP has to address this gap and need to be designed in a way that without strategic clarity, priorities and guidance in decision making, the model itself can’t work.
Principle 35: Intelligent IBP provides clear guidance to make strategically aligned decisions and improves the vertical integration of strategy, planning & execution
Gaps & decision repository: Gaps to plan are important in IBP. Creating a rolling forecast on EBIT level and understanding gaps & upportunities against the mos timportant P&L lines is a must to make gap closure decisions. Somebasic gaps closures can be addressed automaticaly. Augmented (human centric) gap closure decisions that can’t find a resolution are centrally stored and visible at any point in time for IBP stakeholders during an IBP cycle. Plans, gaps & risk are live, transparent at any time and can’t be functionally or organizationally hidden!
Principle 36: Intelligent IBP maintains a centralized, transparent library of planning outcomes, gaps and risks for decisions that require executive attention (this can be an outcome of principle 30,32).
Auditable decision workflow: Cross functional augmented decisions are supported by a smart workflow, where changes in decision policies and decisions over time are visible and auditable (think blockchain). Anything in the decision repository that can’t be solved, is automatically the input for the IBP meeting (feeds in to principle 36).
Principle 37: Intelligent IBP uses a smart, auditable workflow to sign off augmented decisions.
IBP success criteria: traditional IBP often uses supply chain metrics of success. Things like forecast accuracy, DIFOT, inventory holdings, schedule adherence. I believe these are too narrow and a reflection of supply chain bias in IBP in general.
As IBP ought to be a business wide process, better metrics are those of business success like market share/growth, margin, innovation, costs (depending on your strategic intent). Planning specific success can be EBIT accuracy (a measure I had in my KPIs as IBP manager) or cash flow accuracy.
Both supply chain or business wide measures of success, assume that our IBP plans can be executed. However, this is a big assumption & dependency. What if we’re poor in execution?
If we focus on IBP as a decision making forum, we can add measures of success like, decision speed, decision quality, decision impact & resources/time spent to facilitate decisions. Indeed, how many man hours do we use to run an IBP cycle and make decisions? What’s the total cost of ownership of the supporting systems? These metrics remain mostly in the sphere of control of IBP. They are much needed additions, not replacements of other business wide success metrics.
Principle 38: Intelligent IBP success metrics include decision speed, quality, impact and resource need.
Decision communication: I’ve highlighted in detail how IBP decision communication that is aligned with the strategy improves strategy execution, but also improves employee satisfaction and engagement.
Principle 39: Intelligent IBP provides a library with decision outcomes for employees and automatically communicates when new decisions have been made.
Engagement: I’ve long proposed to measure the employee engagement/satisfaction with the IBP process. If we believe IBP is a good thing and ‘the way to run the business’, we should be positive about it right? Well then let’s measure it across all functions! I’ve suggested an IBP Net Promotor Score, but I’ll refrain from adding this as a principle as it might not be specific enough.
If we try to change IBP into a decision centric planning philosophy, covering all decision types in every business planning cycle, we will need to get some employee feedback on the success of our efforts.
Principle 40: Intelligent IBP measures employee satisfaction with ‘the way decisions are made in the business’, and ‘how they are communicated’.
People, mindset & behaviours
Even when we 100% automate (not likely) decisions in the shorter horizon, a human still has to maintain the machine, provide direction, polices and goals. AI without knowing what to solve can be rather dumb.
So, even in automation the human is still a leading factor. With augmentation the human still makes the decisions, and with 100% human centricity (decision about values or behaviours for example), the human is absolutely leading, and the machine is pretty much out of the loop of making the decision. At best will provide augmented analytics. Hence, core principle 3
Accountability: This also means the human needs to stay accountable for the decision, as the human guides the boundaries and targets in which the machine automates tasks and makes decisions.
Principle 41: Intelligent IBP requires the human to be held accountable for the (planning & executional) decisions the machine makes
Mindset: The guidance of a machine, the collaboration with a machine and taking accountability for the decisions of a machine will require a new mindset. One that I’m afraid has not been tested at a large scale. We’re all friends with our mobile phone, but can we become good peers with a machine?
My own IBP mindset surveys tell us that 29% of practitioners think that mindset & behaviours are addressed enough in their organisation. In my field research, 42% of participants answered “Yes” to the question ‘Team members pro-actively engage across all business functions to solve issues.’ We’ll have to work on a better mindset to work with the machine.
Principle 42: Intelligent IBP requires a mindset that supports effective working and collaborating with a machine as a peer or subordinate
As Garry Kasparov says “This is a new form of collaboration where we recognize what we’re good at and not interfere with machines where they’re superior, even if it hurts our pride.”
Collaboration: IBP assumes collaboration between functions, which is unlikely to be present in all organizations, as about 30% of people naturally collaborate and 50% are selfish. Unless a company has collaboration integrated in their recruitment policy, reward and recognition and performance management, it is unlikely it has a critical mass of collaboration, and hence can’t run an effective IBP.
Principle 43: Intelligent IBP uses pre-negotiated cross functional boundaries to replace routine collaborative decisions
Consensus: The ‘Agreement trap’ is a mega bias that confuses agreement with a good decision. Unfortunately, it is incorporated in IBP by design. There is a high focus on reaching consensus in meetings where the IBP teams advocate their recommendations and executives skillfully drill down in the detail through questioning, often without an explicit focus on decision quality. Without rigorously challenging the quality of a decision, we might end up with a consensus as defined by Margaret Thatcher; “The process of abandoning all beliefs, principles, values and policies in search of something in which no one believes, but to which no one objects”.
Principle 44: Intelligent IBP uses pre-defined decision structures with an integrated consensus approach for human centric decisions
Emotional competence: Back in 2014, I defined emotional competence as the final, or highest achievable phase of IBP maturity. In every IBP meeting we need to get the best value out of the discussions and decisions we make, we need to manage different views and conflict to get the best outcome for the business. If a critical mass of leaders have emotional self-awareness, self-control, actually enjoy settling disputes, provide constructive feedback, show empathy for the other silo, develop relationships outside their collaborative teams, build trust, there is a solid foundation to make good decisions. I described this in detail in an IBP behavioral coaching guide.
This is no different for intelligent IBP, however important enough to highlight for high impact, human centric decisions.
Principle 45: Intelligent IBP assumes a critical mass of emotional competence to make high impact, unbiased, human centric decisions
Commitment: Predefined decision protocols, that speed up decision making, reduce analytics resource requirements and reduce human bias can only be effective when a leadership team sticks to those protocols consistently. Guiding a machine purposely to make wrong or ineffective decisions could do a business tremendous harm.
Principle 46: Intelligent IBP requires commitment to agreed decision processes, protocols and working with the machine.
New AI-driven IBP operating models
There is not one simple, straight answer to what the operating model of a highly automated, augmented, AI driven intelligent IBP organization looks like. Especially not for large global, traditional, non-digital native companies that have to transform into a more digital company.
But it’s certain that new IBP models will emerge. That’s simply a matter of time. And it will be more automated, continuous, concurrent, probabilistic, decision centric and intelligent.
My first iteration of a reimagined IBP in the age of AI describes the below schematic in detail and covers many of the principles described.

This will evolve as more analysts, consultancies and vendors start to suggest IBP model adjustments:
Gartner (Dec 2022) started to pay attention to decision centricity in a Decision-Centric Planning blog.
BCG (July 2023) discussed an AI enabled IBP, although I couldn’t decipher what they actually meant with that.
Deloitte (Dec 2023) highlights that the IBP monthly cycle is so passé, arguing for a more continuous IBP, referencing my article.
More (some marketing BS, some useful) ideas will follow and the concept of intelligent IBP using many of the principals discussed here will evolve.
Feel free to join and share your principles or your vision of a future IBP. I’ll keep maintaining this post with new ideas I come across.
Image: Created with DALL-E – “A man on a very high mountain cliff jumping into space digital art”
2 thoughts on “The Intelligent IBP Manifesto – key principles for a new planning paradigm”