An introduction to AI Capacity Planning in Manufacturing
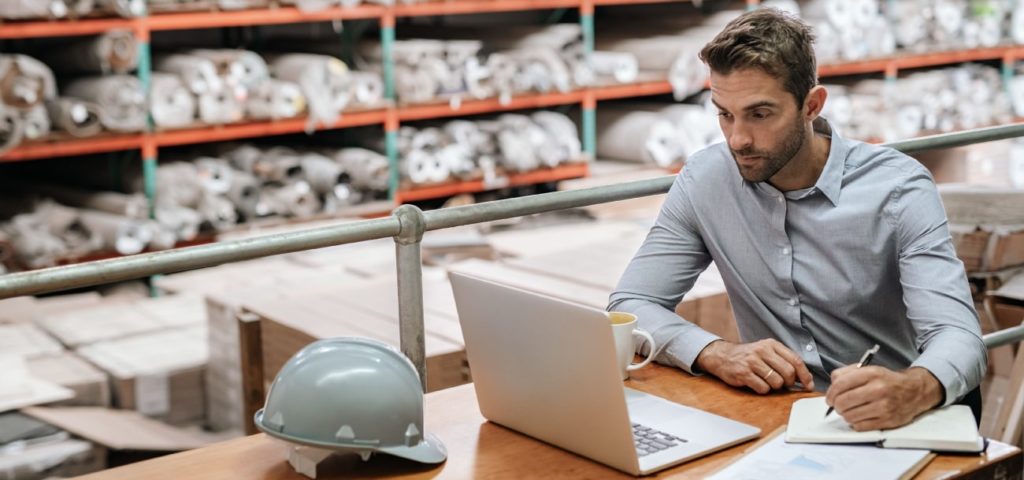
Manufacturing companies often face significant challenges in accurately predicting and meeting demand.
This article explores the transformative benefits of using AI in capacity planning, providing insights into how AI-driven techniques can enhance accuracy, optimize resource allocation, and improve operational efficiency.
We will also dive into how AI-driven capacity planning works, the steps to integrate AI solutions and real-life examples of manufacturing companies that have successfully implemented AI to revolutionize their operations.
Read on to discover how AI can help your business overcome capacity planning challenges and achieve greater efficiency and profitability.
What is Capacity Planning?
Capacity planning is the strategic process of determining the production capacity needed by an organization to meet changing demands for its products or services.
The goal of capacity planning is to balance the supply of resources with demand, minimize costs, and maximize efficiency.
Capacity planning involves resource allocation, production scheduling, and demand forecasting to optimize costs, enhance performance, and improve customer satisfaction.
Accurate resource allocation ensures all necessary resources are available, while production scheduling creates detailed plans for resource use. Demand forecasting analyzes historical sales data and market trends to predict future demand.
Effective capacity planning helps to avoid costs associated with overproduction or underproduction, improves operational performance, and meets customer expectations, leading to increased loyalty and repeat business.
How is AI Used in Capacity Planning?
AI is used in capacity planning to enhance capacity planning through advanced techniques like machine learning, deep learning, NLP, robust data analysis, and automation.
Though we’ve written a comprehensive article on capacity planning strategies, here’s a short overview of AI use cases in capacity planning:
AI Techniques
AI algorithms rely on historical data to learn patterns and predict future resource requirements, providing businesses with foresight for fluctuations in demand.
Utilizing neural networks with many layers, deep learning can model intricate relationships between data elements to forecast more complex patterns. This makes it well-suited for analyzing sizable data collections that normal prediction approaches cannot easily handle.
Lastly, NLP enables sophisticated data differentiation and analysis for unstructured data types like maintenance logs, customer feedback, and marketplace reports for better capacity needs insights.
When coupled, these tools can provide a diverse lens into ever-changing capacity demands for allocations that increase optimal production outcomes for impacted businesses.
Data Analysis
AI excels at analyzing large and complex data sets to generate actionable insights for capacity planning. Processing vast amounts of data from various sources enables AI to identify patterns and trends that inform capacity decisions.
For instance, AI can analyze sales data, production schedules, and market trends to accurately forecast future demand.
This data-driven approach ensures that capacity planning is based on real-time information, reducing the reliance on assumptions and improving the accuracy of predictions.
Automation
One significant advantage of using AI in capacity planning is the automation of the analysis and decision-making process.
AI systems can continuously monitor data and adjust capacity plans in real-time. This dynamic capability allows businesses to respond quickly to changes in demand, production issues, or supply chain disruptions.
Automation reduces the manual effort required for capacity planning, minimizes human error, and ensures that decisions are made based on current data.
What are the Benefits of Using AI in Capacity Planning?
The benefits of AI in capacity planning are improved accuracy, optimized resource allocation that reduces costs, faster decision-making process, and strategic advantages.
Here’s an overview of the benefits of AI capacity planning:
Increased Accuracy
AI significantly enhances the accuracy of capacity planning by processing large and complex data sets and learning from historical and real-time data.
Traditional capacity planning methods rely on static models and historical data, leading to inaccuracies due to changing market conditions and unforeseen variables.
AI continuously updates its models with new data, allowing for more precise and timely predictions. This dynamic approach reduces the margin of error in capacity planning, ensuring that businesses can better align their resources with actual demand.
Resource Optimization
AI algorithms analyze various factors, such as production schedules, workforce capabilities, and inventory levels, to determine the most efficient allocation of resources.
This optimization helps reduce costs associated with overproduction, underutilization, and excess inventory by ensuring that resources are used where they are most needed.
Speed and Efficiency
AI accelerates the analysis and decision-making process in capacity planning. Traditional methods can be time-consuming and labor-intensive, often requiring manual data entry and analysis.
AI automates these processes, quickly analyzing vast amounts of data and providing real-time actionable insights.
The gained speed allows businesses to respond more rapidly to changes in demand, production issues, or supply chain disruptions. The increased efficiency saves time and enables more agile and responsive capacity planning.
Innovation and Insights
AI can uncover new opportunities and solutions that may not be apparent through traditional analysis.
Since AI leverages advanced analytics and machine learning, it can identify patterns and trends humans might overlook.
These insights can lead to innovative strategies for capacity planning, such as identifying new market opportunities, optimizing production processes, or improving supply chain resilience.
AI-driven insights provide a competitive edge, enabling businesses to make more informed and strategic decisions.
How to Integrate AI Capacity Planning Software?
AI capacity planning software is integrated into a four-step process that starts with defining objectives and metrics, collecting and preparing data, selecting the AI capacity planning software solution, and deploying and monitoring the AI solutions.
Here’s a breakdown of each step:
STEP 1: Define Objectives and Metrics
The first step in integrating AI capacity planning software is clearly defining your objectives and metrics.
This involves identifying what you aim to achieve with AI capacity planning, such as improving forecast accuracy, optimizing resource allocation, or reducing operational costs.
You should also establish specific metrics to measure success, such as forecast error rates, resource utilization rates, and cost savings.
Additionally, it is important to outline any constraints and assumptions that will guide the AI implementation, such as budget limitations, data availability, and operational requirements.
STEP 2: Data Collection and Preparation
You must gather data from various sources, including historical sales data, production schedules, inventory levels, and external factors like market trends and weather conditions.
Once collected, the data must be cleaned to remove any inaccuracies or inconsistencies.
This involves data validation, normalization, and transformation to ensure the data is in a suitable format for AI analysis.
Proper data storage solutions should also be implemented to facilitate easy access and retrieval for ongoing analysis.
STEP 3: Choosing and Training AI Models
The choice of AI techniques and algorithms depends on your business’s specific needs and characteristics.
Common demand sensing techniques include machine learning algorithms for pattern recognition and predictive analytics and deep learning models for more complex data relationships.
Once the appropriate models are selected, they must be trained using the prepared data. This involves feeding the data into the models, adjusting parameters, and validating them to ensure they provide accurate and reliable predictions.
Continuous learning and adaptation are also important to keep the models up-to-date with new data and changing market conditions.
STEP 4: Deployment and Monitoring
The final step is to deploy the AI capacity planning software and integrate it with your existing systems.
This involves implementing the AI models into your operational workflows and ensuring they can interact seamlessly with other software tools, such as ERP and CRM systems. Once deployed, continuously monitoring the performance of AI solutions is crucial.
Monitoring includes tracking key performance indicators (KPIs), evaluating the accuracy of predictions, and making necessary adjustments to improve performance.
Regular updates and maintenance are also required to ensure the AI models remain effective and aligned with your business objective.
What are Real-Life Examples of Manufacturing Companies Using AI in Capacity Planning?
Real-life examples of manufacturing companies using AI capacity planning are the BMW Group and General Electric.
To provide additional context, here’s an overview of their challenges, the solutions they’ve implemented, and the results achieved.
Case Study 1: BMW Group
BMW Group, a leading automotive manufacturer, faced challenges maintaining production efficiency and quality control across its numerous manufacturing plants.
A significant hurdle was the complexity of managing production schedules, resource allocation, and quality assurance in real-time.
SOLUTION:
BMW implemented AI-driven capacity planning solutions to optimize its production processes. AI systems analyzed real-time data from production lines, including machine performance, workforce availability, and inventory levels.
Machine learning algorithms helped predict potential bottlenecks and resource shortages, allowing for proactive adjustments.
RESULTS:
The integration of AI led to a 20% increase in production efficiency and a significant reduction in downtime.
AI-driven predictive maintenance minimized unexpected machine failures, while automated quality control systems improved defect detection rates.
BMW achieved better resource utilization, reduced operational costs, and enhanced product quality.
Case Study 2: General Electric (GE)
General Electric (GE) faced challenges in managing the production capacity of its diverse manufacturing operations, which include everything from jet engines to medical devices.
The complexity of coordinating production schedules and resource allocation across different product lines was a significant issue.
SOLUTION:
GE adopted AI-driven capacity planning solutions to streamline its operations. AI algorithms analyzed data from various sources, including production schedules, machine performance, and supply chain logistics. The AI system provided real-time recommendations for optimizing resource allocation and production schedules.
RESULTS:
Implementing AI led to a 15% increase in overall production efficiency and a 10% reduction in operational costs.
GE was able to better align its production capacity with market demand, reducing the risk of overproduction and underutilization of resources.
The AI-driven insights also helped GE improve its supply chain resilience and responsiveness to market changes.
AI Capacity Planning with Throughput
ThroughPut’s ELI, an AI-powered capacity planning software, functions as a bottleneck elimination engine that evaluates your real-time process data. It continuously detects, examines, and addresses bottlenecks to optimize capacity planning.
ELI enables you to overcome operational constraints and maintain a competitive edge by accurately tracking and tracing your manufacturing operations.
ELI provides a comprehensive view of your critical resources, helping you achieve your capacity utilization goals and minimize losses by aligning demand with real-time production needs.
Here’s how ELI supports your operations:
- Resource and Product Views: ELI offers detailed insights into your resources and products, allowing you to track actual, suggested, and available capacities and quantities effectively.
- Comprehensive Overview: It provides a bird’s eye view for capacity planners, operations managers, and chief supply chain officers, enabling accurate estimation of asset load capacity, planning for maintenance, and gaining multi-site and multi-plant visibility for work orders.
- Inventory Management: ELI keeps you informed about inventory levels by tracking and tracing products across the supply chain and assessing changes in market demand.
- Maximizing Real-Time Demand: ELI works to reduce losses by maximizing real-time demand and ensuring resource utilization stays within set capacity limits.
Discover how ELI can revolutionize your manufacturing business. Schedule a demo with the ThroughPut team and experience the transformative impact!
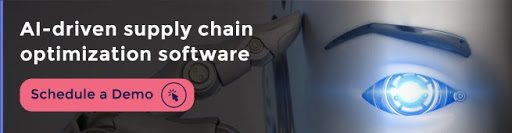