Essential Guide for Demand Forecasting in 2024
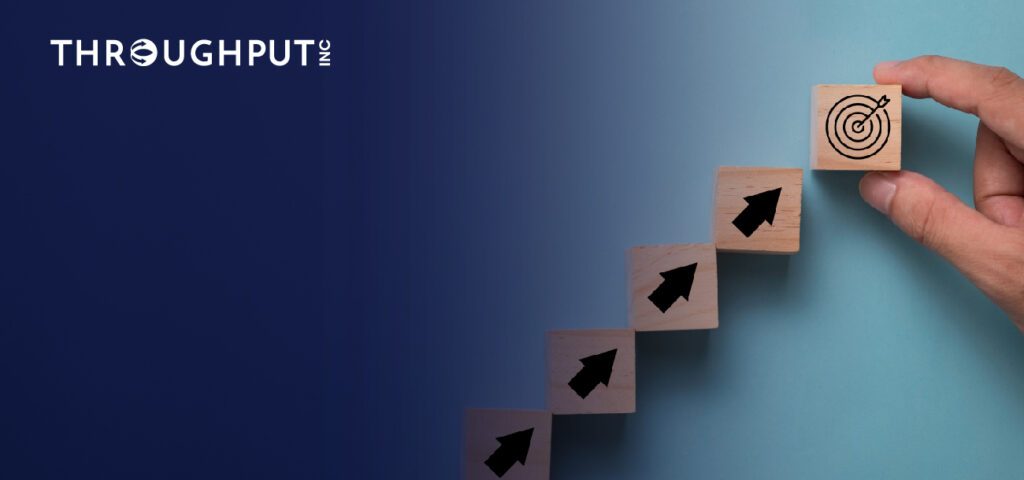
Demand forecasting is a critical component of supply chain management, especially in the complex and unpredictable market landscape of 2024.
Accurate forecasts are essential for maintaining inventory balance, ensuring customer satisfaction, and achieving financial stability. Inaccuracies in forecasting can lead to overstocking, which immobilizes capital, or stockouts, which again result in lost sales and damaged customer relationships.
To address these challenges, supply chain professionals must adopt advanced forecasting methods.
Our 2024 guide provides supply chain managers and planners with the latest tools and insights to refine their demand forecasting processes. Let’s get started with definitions, differences between demand planning and demand forecasting and benefits.
What is Demand Forecasting?
Demand forecasting is the process of predicting future customer demand for a product or service. This practice has evolved significantly from its historical roots, largely a game of educated guesses, to today’s data-driven approach that harnesses the power of advanced analytics.
Demand forecasting is a cornerstone in the modern supply chain, enabling businesses to align their operations with market dynamics and customer needs.
Historically, demand forecasting relied on simple tools and intuition, but as supply chains have become more complex and globalized, the need for sophisticated forecasting methods has grown.
The evolution of demand forecasting mirrors technological advancements and the proliferation of data analytics, transforming it into a precise and strategic function that influences a wide array of business decisions, from production planning to financial management.
What are the Benefits of Demand Forecasting?
The benefits of demand forecasting are enhanced operational efficiency, improved supply chain resilience, reduced excess inventory, and easier identification of new market opportunities all of them lead to increased profits.
The main benefits of accurate demand forecasts are:
1. Improved Supply Chain Resilience
Anticipating demand fluctuations allows companies to adjust their inventory levels proactively, thereby reducing the risk of stockouts or overstocking.
This foresight enables businesses to balance supply and demand, ensuring they can meet customer needs without holding excess inventory that ties up capital and space.
When companies have the right products available at the right time, they avoid the costly disruptions that come with unexpected demand changes, making their supply chains more resilient and adaptable to market dynamics.
2. Easy Identification of New Market Opportunities
Demand forecasting plays a crucial role in spotting emerging trends and market opportunities before they become apparent to competitors.
Analyzing data and identifying patterns allows companies to predict shifts in consumer preferences and market demands, allowing them to innovate and introduce new products or services ahead of the curve.
This proactive approach can open new revenue streams and strengthen market positioning, giving companies a competitive edge.
3. Revenue Increase
One of the most direct benefits of accurate demand forecasting is the potential for increased revenue.
When production and inventory are aligned with anticipated demand, companies ensure they are well-positioned to meet customer needs, maximizing sales opportunities and minimizing lost sales due to stockouts.
Furthermore, accurate forecasting helps optimize pricing strategies and promotional activities, driving additional revenue growth.
4. Reduced Excess Inventory
Accurate demand forecasts mean that companies only produce and store what they need, significantly reducing the costs associated with excess inventory.
This includes the direct costs of storage, insurance, and capital associated with unsold goods and the indirect costs related to obsolescence, depreciation, and waste.
Minimized excess inventory enables companies to operate more leanly and efficiently, freeing up resources for investment in other business areas.
5. Enhanced Customer Satisfaction
Companies can significantly enhance customer satisfaction by ensuring product availability through accurate demand forecasting. Customers expect timely product delivery, and consistently meeting this expectation is crucial for maintaining customer loyalty and trust.
Accurate forecasting helps achieve a high service level, reducing the likelihood of stockouts and the frustration they cause customers.
6. Supporting Sustainable Practices
Accurate demand forecasting contributes to more sustainable business practices by minimizing waste. When producing only what is needed, companies can reduce the environmental impact of overproduction and excess inventory, such as energy consumption, material waste, and emissions.
This benefits the environment and aligns with the growing consumer demand for sustainability, further enhancing a company’s reputation and appeal.
7. Competitive Advantage
Ultimately, accurately forecasting demand is a key driver of competitive advantage. It allows businesses to respond swiftly to market changes, allocate resources more effectively, and make informed strategic decisions.
Companies that excel in demand forecasting can optimize their supply chain operations, reduce costs, increase profitability, and stay ahead of competitors in meeting customer needs while ensuring long-term success and resilience.
What are the Differences Between Demand Planning and Demand Forecasting?
The difference between demand planning and demand forecasting is that they serve different roles within the supply chain. Demand forecasting focuses on the ‘what’ and ‘how much,’ while demand planning addresses the ‘how’ by integrating the forecast with business planning processes.
Demand forecasting predicts future customer demand using historical data and market analysis. On the other hand, demand planning turns these forecasts into actionable plans, considering aspects like inventory levels, production schedules, and procurement.
Demand planning involves collaboration across multiple departments, including sales, marketing, and finance, to create a unified strategy that aligns with the company’s business goals and capabilities.
What are the Types of Demand Forecasting?
The types of demand forecasting are qualitative forecasting, time series analysis, causal models and AI and machine learning models.
Each forecasting type is suited to different scenarios. For instance, qualitative methods may be ideal for new product launches without historical data, while time series and causal models are better for established products with predictable patterns.
- Qualitative forecasting, based on expert opinions and market research. It’s useful when there’s a lack of historical data, but it can be subjective.
- Time series analysis that utilizes historical data to predict future demand. It’s objective but assumes that past patterns will continue.
- Causal models consider external factors, like the economy or competitor actions, that could influence demand. They can be complex but provide a more comprehensive view.
- AI and machine learning models are cutting-edge methods for analyzing large datasets and identifying complex patterns. They require significant computational resources but can offer highly accurate forecasts.
How to Perform Demand Forecasting?
Demand forecasting is performed in a 5-step process that begins with the collection of data and ends with the refinement of forecasts based on real-world outcomes.
This process is about predicting the future and creating a roadmap for businesses, ensuring they meet customer demand efficiently and effectively.
Here’s an outline of each step of the demand forecasting process:
STEP 1: Data Collection
The foundation of any demand forecasting process is the collection of relevant data. This step involves gathering historical sales data, market research findings, and other information that could influence demand, such as economic indicators or seasonal patterns.
The goal is to amass a comprehensive dataset that reflects past performance and current market conditions. This data serves as the raw material for generating forecasts, highlighting the importance of accuracy and breadth in the data collected.
STEP 2: Data Analysis
Once data is collected, the next step is to sift through it to identify patterns, trends, and anomalies. This analysis can be performed using various statistical tools and models designed to make sense of large datasets.
The aim here is to distill the data into actionable insights, identifying underlying demand patterns that can inform future predictions. This phase is critical as it highlights the key factors influencing demand, setting the stage for selecting the most appropriate forecasting model.
STEP 3: Model Selection
Choosing the right forecasting model is pivotal. The selection is based on the insights gained during data analysis, considering the nature of the data, the industry context, and specific business needs.
Models range from simple moving averages for stable demand patterns to complex AI-driven algorithms capable of processing vast datasets and identifying subtle trends. The chosen model should balance accuracy with practicality, ensuring it can effectively apply to the available data.
STEP 4: Forecast Generation
With a model selected, the next step is to generate the demand forecast. This involves applying the chosen model to the data, resulting in a prediction of future demand.
Forecast generation is a phase where the theoretical meets the practical, as the model’s output provides a quantifiable forecast that businesses can use for planning purposes.
The accuracy of this forecast is directly tied to the preceding steps, underscoring the importance of thorough data collection and analysis.
STEP 5: Review and Adjustment
The final step in the demand forecasting process is the review and adjustment phase. This involves comparing the forecasted demand against actual sales data as it becomes available.
Discrepancies between the forecast and reality are analyzed to understand their causes, whether they be changes in market conditions, data inaccuracies, or forecasting model limitations.
Based on this analysis, adjustments are made to the model or the data inputs, refining the forecasting process over time.
Best demand forecasting practices emphasize using a combination of models to account for different market conditions, the importance of regularly updating forecasts with new data, and the involvement of cross-functional teams.
This collaborative approach ensures accurate forecasts reflect a comprehensive understanding of market dynamics.
Additionally, considering external factors such as economic indicators, market trends, and seasonal variations is crucial for capturing the full spectrum of influences on demand.
What are the Best Demand Forecasting Methods for 2024?
The best 5 demand forecasting methods for 2024 are the Historical Data Method, Market Research and Delphi Method, Demand Sensing Method, Predictive Sales Analytics Method and External Macro Forecasting Method.
Here’s a short breakdown of each method:
- Historical Data Method
Historical data method analyzes past sales data to estimate demand during high and low periods. Consider using an AI-powered tool that uses past sales data to make future predictions quickly. - Market Research and Delphi Method
Direct market research and the Delphi method both involve gathering expert opinions and consumer feedback to gain valuable insights into consumer demand. These methods require effort but provide valuable first-hand knowledge.
The success of these methods depends mainly on your available resources and time. Collecting, collating, and analyzing data collected through these methods is time-consuming and will require expertise to leverage the information in predicting market demand.
Again, make it easier by deploying a data-driven tool that collects data from the end consumer and includes local market knowledge.
Blending market anecdotes and insights from professionals obtained from multiple channels boosts forecast precision, expediting the disclosure of useful data for prompt decision-making in a more effortless manner. - Demand Sensing Method
Demand sensing is an efficient method of demand planning that uses machine learning to capture real-time variations in purchase behavior. This helps develop a data-driven supply chain that can quickly respond to unplanned demand changes, improving service levels and forecast accuracy.
In today’s dynamic market, demand sensing powered by AI can provide the most reliable forecasts. ThroughPut AI, for instance, combines internal historic data and external market data to sense demand down to every SKU and location.
This way, you can plan more granularly and accurately without losing critical time in manual data ingestion and insights identification. - Predictive Sales Analytics Method
Predictive supply chain analytics uses ML algorithms and advanced IoT to estimate demand and understand the factors that drive sales. It provides visibility into every step of the supply chain and helps build a demand forecast. - External Macro Forecasting Method
External Macro Forecasting Method examines patterns in the wider economy and assesses their impact on your business objectives.
You gain insights into external market dynamics. Additionally, you can keep track of the availability of essential resources and other factors that may have a direct or indirect impact on your manufacturing processes and overall supply chain.
Similar to previous approaches, artificial intelligence (AI) software can assist in collecting and analyzing relevant data and presenting it in a comprehensive report.
Integrating these demand forecasting methods into a comprehensive demand forecasting strategy will be a key differentiator for businesses seeking to optimize their supply chain and maintain a competitive advantage.
What is Demand Forecasting Software?
Demand forecasting software is an essential tool that automates the complex process of predicting future demand. It leverages various statistical and machine learning algorithms to analyze historical sales data, market trends, and other relevant factors to generate accurate demand forecasts.
The software often includes data visualization, scenario planning, and integration with other business systems, such as ERP (Enterprise Resource Planning) and CRM (Customer Relationship Management).
The latest demand forecasting software goes beyond traditional analytics by incorporating AI to learn and continuously improve over time.
These systems can process and analyze large datasets from diverse sources, including unstructured data like social media posts, to provide deeper insights into consumer behavior.
Demand forecasting software empowers businesses to significantly reduce the time and effort required to produce forecasts while increasing their accuracy and reliability.
This enables more effective decision-making, leading to substantial cost savings and increased customer satisfaction.
What are Demand Forecasting Challenges?
Demand forecasting challenges are data quality and integration, adapting to market volatility, and incorporating new data types.
Despite advancements in technology, businesses continue to face significant demand forecasting challenges. A key issue is the quality and integration of data. As the amount of available data grows, ensuring its accuracy and consistency across different systems becomes increasingly challenging.
Inaccuracies or incomplete data can lead to flawed forecasts, resulting in substantial disruptions within the supply chain. Moreover, market volatility poses another significant challenge. Swift shifts in consumer preferences, unexpected events, and economic changes can quickly make current forecasts outdated.
Organizations need to create adaptable forecasting models that can respond to such uncertainties. Furthermore, integrating new types of data, like sentiment analysis from social media, demands advanced analytical tools and expertise to convert into useful forecasts.
Overcoming these challenges requires a combination of advanced technology, skilled personnel, and robust processes that can adapt to the dynamic nature of the market.
What are the Factors Influencing the Customer Demand Life Cycle?
Factors such as market trends, economic conditions, seasonality, and competitor actions influence the customer demand life cycle.
These factors can significantly impact the demand for products and services. Understanding them is crucial for companies to accurately forecast demand and adjust their supply chain strategies.
Here’s a breakdown the top 4 factors:
Factor 1: Market Trends and Shifts in Consumer Behaviors
Market trends play a pivotal role in shaping consumer demand. These trends can emerge from shifts in consumer preferences, technological advancements, social movements, or consumer behaviors.
For instance, the increasing awareness of environmental issues has led to a surge in demand for sustainable and eco-friendly products. Companies that must recognize and adapt to these trends risk losing market share to competitors more attuned to consumers’ evolving preferences.
Staying ahead of the competition requires businesses to continuously monitor market trends and be prepared to pivot their product offerings and marketing strategies to align with consumer demands.
Factor 2: Economic Conditions
The overall economic environment plays a crucial role in influencing consumer demand. In times of economic prosperity, consumers are more likely to increase their spending on non-essential goods and services.
Conversely, during economic downturns, consumer spending tends to contract, with a shift towards saving and prioritizing essential purchases.
Companies must remain vigilant to changes in the economic landscape and adjust their inventory and marketing strategies to reflect the current economic conditions.
Factor 3: Seasonality
Many products and services experience seasonal demand patterns that vary significantly throughout the year. Understanding and anticipating these seasonal fluctuations is essential for effective inventory management and marketing.
For example, retailers often experience a spike in demand during the holiday season, while demand for travel and tourism services may peak during summer. Companies must plan their production, inventory, and promotional activities to align with these seasonal trends to maximize sales and minimize excess inventory.
Factor 4: Competitor Actions
Competitors’ actions can also influence the customer demand life cycle. New product launches, pricing strategies, and marketing campaigns by competitors can attract consumers’ attention and shift demand away from a company’s products.
Staying informed about competitors’ activities and being ready to respond with innovative products, competitive pricing, and compelling marketing is crucial for maintaining and growing market share.
Understanding these factors is crucial for creating accurate forecasts. Companies must continuously monitor the market and adjust their forecasting models to reflect changes in these variables.
What are the Common Issues with Demand Forecasting?
Common issues with Demand Forecasting are lack of understanding the complexity of demand patterns and data quality and integration issues. These forecasting issues often stem from a combination of factors related to data management, analytical processes, and organizational structure.
Lack of Understanding the Complexity of Demand Patterns
A common pitfall for many companies is an inadequate grasp of the complexity inherent in demand patterns. Various factors, such as seasonal variations, market trends, consumer preferences, and external events like economic shifts or global crises shape demand.
Companies may need help incorporating these multifaceted influences into their forecasting models, resulting in predictions that do not accurately reflect market realities.
- Seasonality and Trends
Companies may need to fully account for the nuanced patterns of seasonality or recognize emerging market trends. For example, a retailer might underestimate the surge in demand for certain products during holiday seasons or not anticipate a growing consumer preference for eco-friendly products, leading to either stockouts or overstock situations. - External Events
Natural disasters, economic downturns, or pandemics can drastically disrupt demand patterns. Companies that incorporate flexibility and scenario planning into their forecasting processes may need to prepare for such disruptions, leading to operational and financial difficulties.
Data Quality and Integration Issues
Another prevalent issue is the quality and integration of data used in demand forecasting. High-quality, relevant data is the foundation of accurate forecasting.
Still, companies often need help updating, completing, or siloing across different departments, leading to forecasts based on an inaccurate or incomplete picture of demand.
- Data Silos
Data fragmentation within organizations can prevent a comprehensive view of demand drivers. For instance, if marketing data regarding upcoming campaigns is kept from the supply chain team, the potential increase in demand may need to be factored into the forecasting model. - Data Quality
The accuracy of forecasts heavily depends on the quality of the underlying data. Companies often need help with inaccurate or not granular enough data to provide meaningful insights, such as sales data that does not account for returns or cancellations, leading to an overestimation of demand.
How to Improve Demand Forecasting?
Improving demand forecasting involves mitigating the mentioned common issues, resulting in improved accuracy and reliability of demand forecasting.
Companies should take several recommended approaches:
- Enhance Data Collection and Integration
Implementing integrated data management systems can help break down silos and ensure that all relevant data is captured and shared across departments. This includes internal sales and operations data and external data such as market trends and economic indicators. - Incorporate Advanced Analytics and AI
Advanced analytics and AI technologies can help companies better understand complex demand patterns and predict future trends more accurately. Machine learning models can analyze vast datasets and identify patterns that may not be apparent through traditional analysis. - Flexibility and Scenario Planning
Building flexibility into the forecasting process and regularly conducting scenario planning can prepare companies for unexpected changes in demand. This involves creating multiple forecast scenarios based on market conditions and consumer behavior assumptions. - Continuous Monitoring and Adjustment
Demand forecasting should be a continuous process that regularly monitors actual demand versus forecasted demand. This allows companies to quickly identify discrepancies and adjust their forecasts and supply chain strategies accordingly.
Mitigating these issues involves fostering a culture of collaboration, integrating data sources, and staying attuned to market changes.
Companies must also embrace new technologies and methodologies that can enhance the accuracy of their forecasts, such as machine learning algorithms that can detect patterns not evident in historical data alone.
How does Demand Forecasting Affect Supply Chain and Fulfillment?
Demand forecasting affects supply chain efficiency and fulfillment by ensuring that the right products are available at the right time, reducing the risk of stockouts or excess inventory, and optimizing distribution and logistics operations.
The ripple effect of accurate forecasting is felt throughout the entire supply chain. It enables better capacity planning, more efficient use of resources, and improved customer service levels.
How does AI Software affect Demand Forecasting?
AI software can positively affect demand forecasting as it can process and analyze large volumes of data, learn from trends, and make predictions with a high degree of accuracy.
Firstly, the use of AI permits the analysis of intricate patterns in vast datasets, which would be beyond the abilities of human capabilities to obtain more dependable results.
Secondly, companies that use AI software can analyze data efficiently in real-time, resulting in rapid responses to changes in market studies.
Special insights are another verge of automation since, compared to individualistic scrutiny techniques, AI systems incorporate predictive insights for future activities and tendencies in the industry.
AI is also complemented with rollout; novel datasets and supply chain networks present no obstacles, resulting in scalability. The industry is competent by AI-based demand forecasting, which enhances decision-making, and costly ventures can evolve to bring efficient savings.
How to Integrate AI software into the Demand Forecasting Process?
Integrating AI software into the demand forecasting process involves several five key steps starting with data preparation, choosing the AI software solution, system integration, training and testing demand planners and continuously learning.
Integrating AI into demand forecasting begins with the meticulous preparation of data, a crucial first step that involves the cleaning, normalization, and structuring of data gathered from diverse sources, including sales records, market research, and external data streams.
Following this, the selection of an AI software solution is paramount. The chosen software must not only meet the company’s specific requirements but also possess the capability to manage the data’s volume and complexity while offering the necessary analytical tools for effective demand forecasting.
The integration process extends to ensuring that the AI software is seamlessly incorporated into the company’s existing IT infrastructure, including ERP and CRM systems. This integration is vital for facilitating uninterrupted data flow and ensuring data is readily accessible for analysis.
Furthermore, the demand planners play a critical role in this process. Before the AI system is fully deployed, it must undergo a training phase using historical data, followed by rigorous testing to verify its ability to accurately predict demand patterns.
An essential feature of AI software in demand forecasting is its capacity for continuous learning and improvement. As the system is exposed to new data and as market conditions evolve, the AI is designed to adapt and refine its predictions, thereby enhancing its accuracy and reliability over time.
This ongoing learning process is critical for maintaining the effectiveness of demand forecasting in a dynamic market environment.
What are the Future Trends of Demand Forecasting?
Future trends in demand forecasting are the increased use of AI and machine learning, the integration of real-time data streams, and the development of more collaborative forecasting platforms.
These advancements will enable even more precise demand predictions and supply chain optimization.
As we look to the future, AI-based demand forecasting is set to expand further, with developments in areas such as deep learning and neural networks offering even greater analytical power.
The integration of IoT devices and the increasing availability of big data will also play a significant role, providing a continuous feed of real-time information that can be used to refine forecasts and respond to market changes instantaneously.
Demand Forecasting with Throughput
ThroughPut AI software is powered by artificial intelligence and excels in enhancing supply chain planning and management through its adept demand forecasting and sensing capabilities.
This solution is equipped with a sophisticated Demand Sensing Module. This module leverages cutting-edge algorithms and machine learning to craft precise forecasting plans, suggest ideal inventory levels, and formulate operational strategies that resonate with current market dynamics.
When utilizing the Demand Sensing Module, you gain the ability to accurately anticipate upcoming demand trends, adjust supply sources to minimize waste, and efficiently manage the movement of materials and processes. This, in turn, helps optimize cash flow throughout your supply chain.
Rather than relying solely on past data, the software incorporates a variety of external variables, including economic trends, geographical factors, and environmental conditions, into its analysis.
These elements are analyzed in real-time, together with demand-driven insights, to produce easily interpretable results.
Book a demo to find out how to accurately forecast demand with Throughput.