We are in the middle of a hype cycle. The acronym for artificial intelligence (AI) is seemingly everywhere, but the value proposition is nowhere. Like any hype cycle, the market will overheat being over-hyped, then fall. The question in my mind is, “Will we drive substantial improvement in outcomes? Or transform work?” What I see today, I find disappointing. How about you?
Before reading this post, consider reading my prior blog post, Don’t AI This.
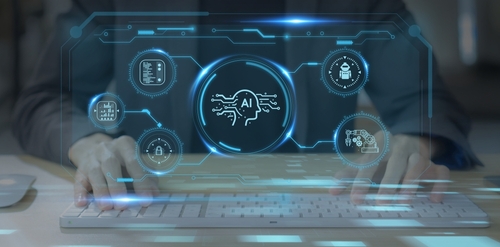
Let’s Start With Clear Definitions
My inbox is full. The market is flooded with references to AI, but few stop to give a definition. To combat the hype cycle to drive value, I think that we need to get clear on use cases and definitions. But before we start, let me give you the caveat that I am not a technologist. I am a researcher. So, feel free to correct me or join/elevate the discussion on the definitions or use cases. Let’s have fun!
Let’s start with the definitions:
- Graph AI uses Machine Learning on graph-based technology to understand the relationships between variables to drive insights. Graph technology leverages graph structures to represent and store data. (Conventional solutions operate on relational databases with a predetermined schema.) By using techniques like clustering, partitioning, and shortest path, some problems become easier to solve. These include problems where centrality, connectivity, and path analysis play a key role in the analysis.
- General AI is a self-learning form of artificial intelligence based on language processing to learn across systems. Chatbots are often a delivery mechanism for general AI.
- Narrow AI is goal-orientated Artificial Intelligence designed to solve specific issues, such as determining stock levels or planning promotions based on events or weather. The design is a specific use case. Many of today’s solutions include Narrow AI.
- Artificial Superintelligence. This level of AI is theoretical and speculative. Great to conceptualize potential use cases, but not today’s reality.
Use Cases
I think that the use cases for Narrow AI to improve insights are pretty clear. We have this today in most supply chain planning solutions. I don’t think that the answer is faster planning or better engines. Instead, I think that the opportunity is redefining work. My focus in this post is on the graph and GenAI.
I spoke to a company weeks ago that was excited about using the Sales Force Platform and AWS to improve planning. My question, which was unanswered, was, “How does using a different relational database platform improve the outcomes even if it is fast and in the cloud?” Same for SAP HANA. My Point of View (POV) is that the best use case for SAP HANA is the in-memory capabilities for repetitive work. The problem with supply chain planning is that it is, by definition, not repetitive work. Additionally, the tight integration of ERP to APS (Advanced Planning) makes the system nervous and throws supply chain results out of balance, increasing the bullwhip impact.
We also need to throw away the concept of real-time planning. Planning should never be a real-time process. I find that companies confuse responsive with reactive. Many supply chain leaders are action-oriented and want to drive action. Running optimizers frequently introduce noise and error into a complex system. (Not a good thing.)
So, what can we accomplish using GenAI and the graph? Here are my thoughts:
- Self-service Planning for Use by Business Leaders. I laughed this week at two comments on LinkedIn. The first was that the industry would be short 60,000 planners in five years, and the second was that GenAI is like having a junior planner working in concert with a senior leader. My belief is that GenAI on the Graph, through the deployment of outside-in thinking (freeing ourselves from the limited thinking of convention), offers the opportunity to reduce the number of planners by 80-85%. Forget the concept of a junior planner. I say, “Let’s get busy redefining work. Why do we need hundreds and thousands of planners?” I believe that the focus should be improving reliability (Forecast Value Added (FVA), first pass tender and yield, and manufacturing schedule adherence) while decreasing process, demand, and data latency. (The focus needs to morph from focusing on reducing error to improving reliability. and from a series of labor-intensive meetings that delay decisions to powerful insights for modeling.) As a result, we need to throw away the conventional definitions of demand planning and S&OP. How so, you might say? Let’s start here:
- Data Analysis. We are not listening to what data has to tell us. In today’s processes, we move data into jails (relational databases) and then run optimizers. We only use 20% of the data surrounding the supply chain rejecting anything that does not neatly fit into rows and columns. Gen AI helps us answer the questions we don’t know.
- Knowledge Graph Development and processing at the speed of business. Today’s demand processes analyze shipment and order patterns. The elongation of product platforms to serve micro-markets, increases demand latency. (As product velocity slows, the time to translate shelf takeaway/consumption to order visibility. This time is usually weeks and, in many products, three months.) As a result, the dependence on an order signal puts the organization on a back foot. The supply chain benefits through the use of market data at the speed of markets. Shortening the cycle for demand insights is a powerful use case.
- Sensing and Proactive Alerting. The complexities in a supply chain loom large. The translation of ripple effects of short supply, pricing, quality issues, and freight shifts affects how to source, make, and deliver processes should align. In today’s systems, they act independently based on order signals.
- Adaptive Model Redefinition Through Learning. Today’s systems use fixed hierarchical relationships. As products and markets change, the models need to be adaptive.
- Interoperability Through Bots? Today, we speak of integration. The goal needs to be interoperability. Batch interfaces are slow. Can bots help us to move insights from system to system and user to user? Is it possible to close the black holes of spreadsheets and emails that hold us hostage in the management of networks?
- Brain Drain. I have lived through the evolution of five generations of supply chain leaders. Sadly, I don’t feel that we are standing on the shoulders of giants. My concern is the growing knowledge gap between consultants, system integrators, and business leaders. The discussions are full of vacuous presentations and over-hyped promises. Too few have real-life experience. Sadly I observed that as the training and knowledge sharing over the last two decades became more available, it was also less valuable as the supply chain became more mainstream. I find the industry awash with opinions but short on research-backed answers. GenAI is promising as a means to train and help leaders learn.
- Improve Sensing with Market Data. Today’s supply chains react, but they do not sense or learn. What if we could continually sense and drive role-based insights within the organization? Build customer narratives by mining disparate data and translating it into insights. Or mine supplier shifts to build alternate buying plans. All are possible… However, to make the shift, we need to redefine work.
- Test and Learn. Our processes to understand the market response to products are slow and cumbersome. What if we could intelligently design cross-channel and product portfolio testing and learning scenarios? Instead of relying on focus groups, we could learn through clicks/streams/ratings and reviews and apply the learnings continuously to markets.
So, the next time someone talks to you about GenAI while waving their heads to argue that it helps you through a junior planner-like capability or brags about real-time planning capabilities, challenge the status quo to think bigger. In my opinion, Gen AI should help us redefine work. The supply chain planner has the lowest satisfaction level of any supply chain employee, and 97% of companies lag behind their peer group to manage a balanced scorecard of growth, operating margin, inventory turns, and Return on Invested Capital. Isn’t it time to think beyond today’s paradigms to capture these opportunities?
Meanwhile, I will test and socialize these concepts by teaching open classes with technologists and business leaders. I think that it is time to question the status quo.
I look forward to getting your thoughts.