Data and Supply Chain: Seven Myths That Hinder Your Success with AI and Analytics
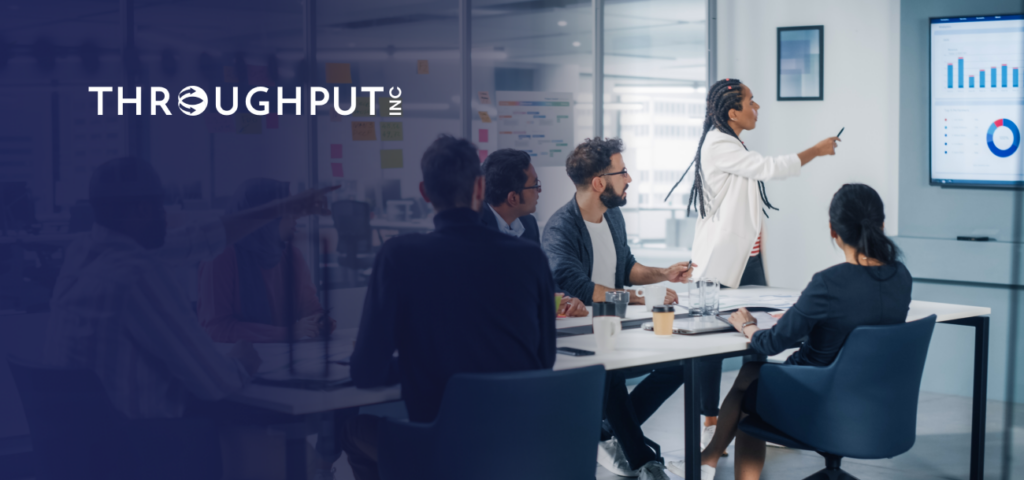
Organizational supply chains are evolving into sophisticated data hubs.
The era of merely transporting products is over. Modern supply chains demand extensive coordination and tracking, generating vast amounts of data—several petabytes, in fact.
By harnessing real-time insights from this operational data, supply chain performance and visibility can be significantly improved.
That said, data is not a new element in supply chains.
Systems like the Manufacturing Execution System (MES) have been producing operational data for decades. Yet, many operations teams struggle to fully utilize this data for digital transformation in the supply chain.
This is largely due to pervasive myths about data utilization, which hinder operational excellence and prevent organizations from achieving their goals.
In this blog, we aim to dispel the prevalent myths surrounding the use of industrial data in supply chains that might be stopping you from investing in AI or deriving value from it.
Remember, your data is powerful—your data is potent.
Take the first step toward leveraging your existing data for remarkable industrial outcomes.
Data and Supply Chain: The Seven Myths You Should Know About
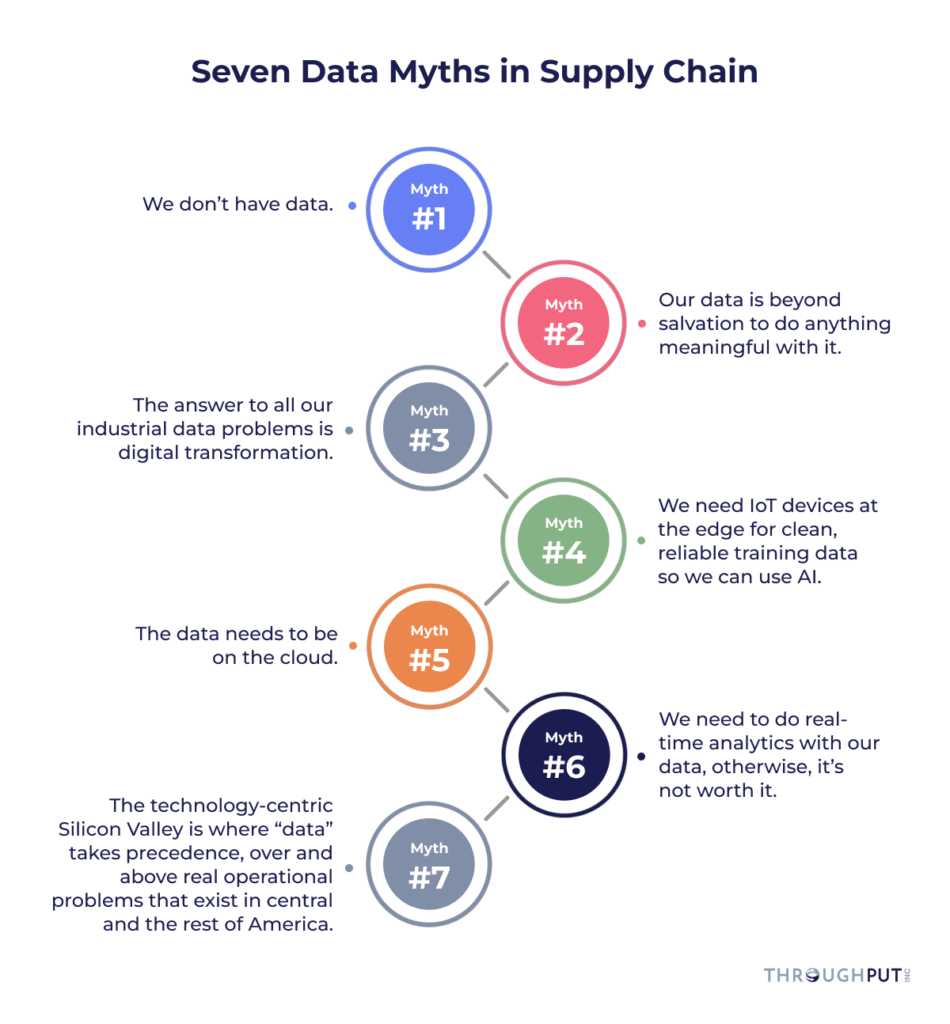
MYTH #1. We don’t have data.
A report by Cisco in 2018 noted that 5 quintillion bytes of data are created every day around the world; your organization being one among them.
Fact Check The worldwide Big Data market revenues for software and services are projected to increase from $42B in 2018 to $103B in 2027.
Perhaps there is no bigger pushback that AI and data analytics companies get from industrial companies than “we don’t have data”.
This is not the fault of the business as they have never been made aware of what exactly is industry operational “data.”
Firstly, let’s decode “data” itself.
When many organizations think of data, they think of real-time data being generated from edge sensors.
While that is 21st-century data, corporations have been generating operational data for centuries.
All the accounting systems, worker time logs, payroll slips, inventory ledgers, bank ledgers – anything with transaction data (a recorded) event is data.
ThroughPut’s Take on This Myth
It is impossible to be a business today and not have data. The IRS wouldn’t allow it.
Regardless of whether organizations are using Excel, SAP, ORACLE, QAD, or a chalkboard measuring quality, data exists and can be leveraged.
It is true that 100% of all companies have data that can be utilized to run better operations. Operations managers just need to figure out where it exists and how they can begin using it.
Did You Know?
Today, many manufacturing companies are using equipment that records vast volumes of sensor data. Unfortunately, these same companies are not putting that data to good use.
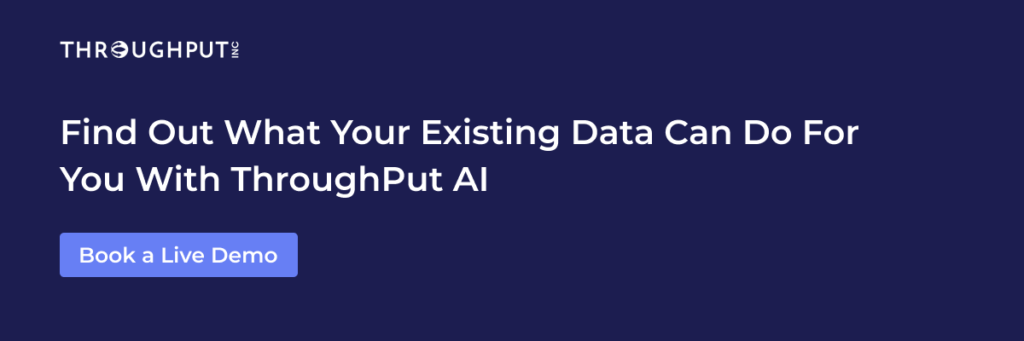
MYTH #2. Our data is beyond salvation to do anything meaningful with it.
Dirty data might exist in your organization but the problem isn’t that, it is the lack of the right tools or the knowledge to address this messy data that is the problem.
Fact Check
- Most organizations do nothing to remedy their data misalignment other than running a periodic clean-up exercise only to find themselves in the same mess a year later.
- According to Forbes, more than 70% of active party data is incorrect, ambiguous, and/or incomplete.
- According to IDC, “Data monetization” will become a major source of revenue with 180 zettabytes of data in 2025, up from less than 10 zettabytes in 2015.
This seems to be the #1 opt-out reason from digital innovation groups, which can be a big mistake.
First, it’s time to tackle this dirty data.
Let’s put things in perspective through an analogy: Crude oil being dirty didn’t stop it from becoming a huge opportunity for the oil industry to commercialize it and take over the global economy.
Similarly, nothing stops dirty data from being used by technology to take it to the next level and derive pure insights from it.
The biggest problem is not the dirty data but the lack of initiative to use the correct tools to address it.
To begin with, corporations must select the right “DNA” of data that is worth the effort to clean. Compared to data scientists, operations people know what data is relevant, because they understand the core of operations.
There is also another big organizational problem simultaneously at play. Many times, digital innovation leaders fail to involve operational leaders in their roadmap to data-driven operations until the implementation.
This is because most operational people expose resource realities, quickly take bold ideas, and repurpose them for practical use.
Digital innovation groups need months to solve today’s problems and operations teams don’t have that kind of time to invest in training for this.
Even though they may be a part of the same company, operations and digital innovation groups focus on different time-frame objectives.
In other words, they don’t share the same pain points.
However, there is a way both of these groups can work together.
Operations domain experts can be leveraged to expedite data cleansing. These experts can point out what data matters, saving weeks of analysis time for data and digital teams.
These insights can ultimately be used by digital teams for their product innovations.
ThroughPut’s Take on This Myth
At ThroughPut, data cleansing is faster as we deploy the best-of-breed algorithms and 42+ heuristics, which makes data cleansing easier and seamless.
Our team of data analytics experts plays a vital role in ensuring this happens consistently.
Therefore, companies should not limit themselves to assumptions about dirty data, but rather figure out ways in which the right data analytics tools can be used.
Did You Know?
- Forbes reveals that 95% of businesses strongly need to manage their unstructured data.
- Forbes also suggests that more than 150 zettabytes or 150 trillion gigabytes of data will need analysis by 2025.
MYTH #3. The answer to all our industrial data problems is digital transformation.
Usually, answers to most industrial problems lie in the existing, deep-seated data, processes and knowledge capital.
Fact Check
- According to Forbes, Digital Transformation is a 1.3 Trillion-dollar industry. Surprisingly, Forbes also found that 84% of companies fail at being digitally transformed.
- If global corporations collectively lose $1 Trillion on digital transformation efforts, is the path to data-driven operations more digital transformation?
While it may seem otherwise, the real answers to industrial data problems can be found within the existing data systems and people’s expertise, which are most often siloed.
With the right mix of a data-driven culture and the use of relevant data analytics tools, organizations can benefit from this existing data.
Digital transformation makes sense for corporations that already have engineers who have spent decades manually optimizing their operations by pulling data and pushing it through statistical and visualization tools. They now can no longer extract value from dynamic problems using static tools.
While digital transformation may bring more holistic access to organizational data, nothing is preventing operational teams from tying together existing disparate data to impact bottom lines, worker training and safety, and output today.
ThroughPut’s Take on This Myth
Digital transformation is not a prerequisite to extracting value from industrial data.
The prerequisite is having a culture around using existing tools to solve existing problems regardless of what is going on with existing data
Did you know?
- More than $100 billion is wasted each year on digital and analytics business transformations that do not deliver what they promise.
- McKinsey reports that only 14% of 1,733 business executives polled, said that their digital transformation efforts have sustained performance improvements, with only 3% reporting complete success at sustaining change.
MYTH #4. We need IoT devices at the edge for clean, reliable training data so we can use AI.
IoT devices are not a prerequisite to solving industrial-scale problems- an alignment of mindset is.
Fact check
- This may be news, but industrial operations have had controllers and sensors on their devices for over 75+ years.
- Also, AI has been around for 40+ years.
IoT does generate cleaner data but the real question is whether organizations are in a position to solve problems of scale using Machine Learning or AI.
Industrial operations have had embedded sensors for decades, though the devices used earlier were not as advanced as Nest and Alexa to turn switches on and off.
The road to IoT starts with the analysis of current data and creating a simple approach to intelligent data collection.
At times, for greater visibility within operations, additional IoT sensors can help but are not prerequisites.
Technology isn’t the problem here.
The problem is shifting the mindset of industrial corporations away from the concept of “newer is always necessary.”
This is where corporations really need to slow down and ask a few questions.
- Where do you really stand with leveraging your existing sensor data?
- Are you ready to leverage true AI at an 80 factory-scale, and run AI-powered operations, and is everyone on board with it?
- Are you just doing AI because your competitors are doing AI?
ThroughPut’s Take on This Myth
The real problem lies in not using existing sensor data to meet prevailing operational behavior, before investing in IoT.
At ThroughPut, this has been thought through at a much deeper level. Even with the world’s leading process management and AI thought experts on board, we often provide the litmus test as to organizations to where they actually stand with AI-powered bottleneck elimination from a real operations perspective.
The fact is that ML/AI products like ThroughPut can be leveraged today to help organizations leverage their sensor data, provided it is backed by the right mindset to do it.
Did you know?
- Collecting and transmitting data from industrial machines and devices is not a new concept. Since the early 80s, data from industrial assets has been captured, stored, visualized, and analyzed to improve key business impacts.
- According to our research, only 4% of corporations use AI in their supply chain. Therefore, the actual problem is not IoT and Edge AI.
MYTH #5. The data needs to be on the cloud.
The truth is that the data does not have to be on the cloud to generate insights.
Fact Check
- To date, 95%+ of ThroughPut’s engagements have not been required to pull data from the cloud. Whether the data is on-premise or on-cloud is a corporate preference around storage, not data extraction itself
- The typical technology workforce needs to be reskilled or upskilled for the cloud environment as they are well-versed in developing business applications in the traditional IT framework.
From ThroughPut’s perspective, the cloud makes it so much easier to find, track, and work on operational data and also saves the service costs associated with physically showing up to a factory to pull data.
This is especially true for demanding industries like oil wells where it can be challenging to pull crude oil data physically, but has to be done.
So, while it is not mandatory for data to be on the cloud for insights, it is just more effective for prescriptive and predictive analytics companies like ThroughPut, to make life easier.
The integrations are faster, the throughput is higher, and data ingestion can be controlled for both long-term strategy planning and real-time root cause resolution.
ThroughPut’s Take on This Myth
While data being on the cloud can increase output, profitability, and ultimately the earnings-per-share of the company faster, it is not a prerequisite.
The first step even before that, is to get the right data and treat it appropriately for accurate insights.
Did you know?
- Many enterprises are stuck with supporting both their inefficient traditional data center environments and inadequately planned cloud implementations that may not be as easy to manage as they imagined.
- Today, for many large companies with complex IT architectures, moving applications and data to public cloud platforms involves working through a formidable set of technology, security, operational, and financial issues.
MYTH #6. We need to do real-time analytics with our data, otherwise, it’s not worth it.
This is not mandatory.
Unless your operation is time-critical and has lots of money and lives on the line. Even then, it comes down to your real-time ability to respond to recommendations.
Fact Check
- Gartner claims that 87% of corporations have low business intelligence maturity. That means, 87% of the world’s industrial companies don’t even use basic visualizations available from Tableau, Qlik, Watson, or Excel Power BI plug-ins.
- While everyone around you has been asking for real-time analytics for more than 5 years now, there is a 1 out of 10 chance you actually need real-time analytics.
Real-time analytics is a must in some extreme cases with irreversible consequences like the hydraulic plant where fluctuations in the real-time analytics window can change operational dynamics in a matter of a few seconds.
So, depending on if you are running a dynamic operation, where real-time bottleneck elimination and root cause analysis could save the company millions or lives, then it qualifies for real-time analytics.
If not, then it could be a good idea to look at the factory data from a historical perspective across locations at scale.
The truth is that most customers need exposure to analytics before deploying real-time advanced predictive and prescriptive analytics for their operations.
At ThroughPut, we always evaluate the necessity to deploy these real-time analytics to help the client make real-time operational and financial decisions.
ThroughPut’s Take on This Myth
Depending on the organization’s current stage, this involves leveraging existing data to replay historical time-stamped information, allowing for a comprehensive understanding of the organization’s operational history.
The question every “real-time analytics” buyer needs to ask is, “If I lost my real-time analytics screens right now, would it cost me my job, lives, or lots of money?”
If the answer is yes, it’s time to get procurement involved.
Did you know?
- Using real-time insights requires a different way of working within your organization. It relies on receiving information every second. This might need an actual culture shift to become an information-centric organization.
- Real-time analytics, if not implemented properly, could be a disaster. If a business isn’t used to handling data at such a rapid rate, it could lead to incorrect analysis, which could cause larger problems for the organization.
MYTH #7. The technology-centric Silicon Valley is where “data” takes precedence, over and above real operational problems that exist in central and the rest of America.
The perfect solution can only be arrived at when technology meets operations, with data as the common multiplier.
Fact check
- According to Forbes, many forward-thinking manufacturers are orchestrating 80% or more of their supplier network activity using big data and cloud-based technologies to get beyond the constraints of legacy Enterprise Resource Planning (ERP) and Supply Chain Management (SCM) systems.
- The same study reveals that 64% of supply chain executives consider big data analytics a disruptive and important technology, setting the foundation for long-term change management in their organizations.
In the typical industrial world, there is a huge debate around whether the Silicon Valley technology troop understands what the Middle of America-based operations troops do and what their real problems are.
This is because most of the time operations and technology teams work in silos.
While the operations team perceives “data” to be a purely technology-oriented domain, technology teams often fail to relate to the day-to-day struggles of an operations person.
The fact is that real operations problems on the factory floor will only get resolved when technology teams use “data” to solve operations problems.
For this, it is important to come up with a well-balanced strategy that involves both technological expertise and operational excellence and uses data-oriented analytics to create sustainable, safer, and efficient operations.
This will ensure a win-win for both teams.
Did you know?
- Data plays a strategic role across the operations life cycle – it has an impact on the organizations’ reaction time to supply chain issues (41%), increased supply chain efficiency of 10% or greater (36%), and greater integration across the supply chain (36%).
- Big data analytics embedded operations lead to a 4.25x improvement in order-to-cycle delivery times, and a 2.6x improvement in supply chain efficiency of 10% or greater.
How to Overcome Data Challenges in Supply Chain?
Overcoming data challenges requires companies to understand the difference between good and not-so-good data.
“Good data is useful data. Is good data always entirely 100% correct? No, it doesn’t have to be. Because you can have consistently incorrect data and still use it to find patterns. On the flip side, you can have entirely correct data, but it could be completely useless,” said Genevieve Shattow, the head of analytics at ThroughPut.ai to The Logistics Report.
Good data is not just about accuracy but also needs to be relevant in context to the problem that requires solving.
That means the same data can be good in certain situations and not-so-good in certain conditions. “The importance of data is tied to its situation,” contends Shattow.
She also says, “You need data at the cadence at which you will take action. If data isn’t coming in real-time, it’s not going out in real-time either.” Also, companies that have data flow in real-time are usually not equipped to handle data at such frequency.
An important aspect of overcoming data challenges is to get good useful data and the key to that lies in how companies treat the people who handle this data.
As Shattow says, “Mathematically speaking, errors propagate. If you have uncertainty at the beginning, the only way to decrease that uncertainty is with lots of data. You need someone who understands how to combine data and how to propagate it to its best intent.”
Companies also need to understand the difference between people who design data systems and those who use data.
You need to get a good data engineering team on board but also ensure that you have people who have domain-specific expertise – the ones who really understand data.
As Shattow sums it up, “It’s not just about the data itself, but about how you manage and interpret it that ultimately determines its effectiveness and accuracy in decision-making processes.”
Data and Supply Chain: Making the Best of What You Have
About data and supply chain, it is crucial to point out that effective data leads to optimized supply chain operations.
ThroughPut makes the best use of existing data sets to deliver tangible results right when required.
The tool uses an adaptive approach so that organizations can harness the full potential of their data resources.
It seamlessly works with existing systems like ERP and other legacy systems to utilize their current data infrastructure, eliminating the need for complex data migrations or overhauls.
The tool ingests data from diversified sources, including Excel sheets and other data assets in their native formats too.
Since ThroughPut integrates different systems and works with existing data, organizations do not need to spend too much time in extensive data gathering, data cleansing, or data interpretation to see encouraging ROIs.
No more worries about data not being enough or good enough!
The entire process runs in an accelerated mode. Besides speed, ThroughPut drives accurate data-driven solutions.
With the help of iterative data analysis and pilot programs, ThroughPut helps build AI models based on accurate and validated data.
The tool builds on data slowly, which in turn, mitigates the risks of inaccuracies or biased AI models making all insights and recommendations highly reliable for confident usage.
To summarize, ThroughPut is totally focused on data and supply chain optimization and prioritizes validation and accuracy in AI models.
The tools help organizations make the best of what they have and how much they have, driving innovativeness and efficiency in their supply chain operations.
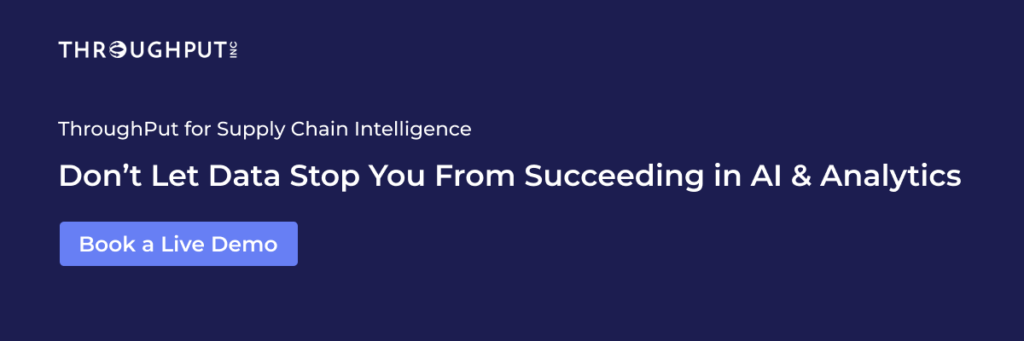