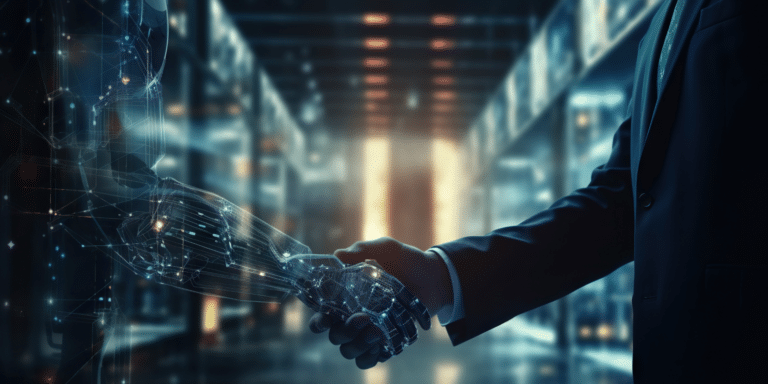
Supply Chain AI has sparked considerable excitement, shock, and fear over the past year. From Generative AI innovations like ChatGPT to industry events, analysts, and mass media stories, every business leader is being tested – from their vision and trust in the technology to internal preparations and integration of artificial intelligence into supply chain operations.
The primary reason for such a range of emotions stems from not knowing how to adopt AI advancements. In one of our recent webinars, a poll showed 76% of attendees were in the educational stage of adopting Generative AI in their companies. In addition, another poll, just one month later showed that 31% of attendees were cited saying they are either developing proposals to begin or currently testing pilots that incorporate AI to their companies.
Considering where most companies are in their Supply Chain AI journey, the mix of excitement and apprehension is no surprise. This phase is often characterized by a range of questions that are challenging to answer, including:
- How can we trust and validate the information Generative AI produces?
- How can our organization best prepare for current and future AI capabilities?
- How can we move forward when internal resources are limited – from planners to data scientists?
To truly overcome this common hurdle to adoption, business leaders must understand the difference between Generative AI and machine learning and know which use cases deliver the greatest-possible impact.
The differences between Generative AI and machine learning
Generative AI and machine learning are closely related within the broader realm of artificial intelligence. However, there are critical differences between the two: their primary objectives and outputs. Unlike machine learning, which is predominantly task-oriented, Generative AI is more about creating original content that doesn’t necessarily relate directly to specific input data but instead learns the underlying structure to produce new, similar results.
Machine learning is a branch that involves algorithms and statistical models enabling computers to improve their performance on a task through experience or data. The technology encompasses various techniques to learn from labeled or unstructured data to predict or classify based on given data, make decisions or inferences, and train models to perform tasks. The system then learns patterns and makes predictions or decisions based on the provided data, essentially focused on specific tasks such as classification, regression, or clustering.
Generative AI, on the other hand, is a subset of deep learning that deals with the creation of new content or data based on both labeled and unlabeled data. This field primarily focuses on creating new content – including images, text, audio, or videos – that may not have been part of the original dataset based on patterns and information learned and recreated from the input data.
Knowing these differences between the two technologies is pivotal for businesses aiming to leverage artificial intelligence effectively. But the complementary relationship between the two must also be acknowledged, especially as advancements in one ultimately benefit the other and contribute to the broader development of sophisticated applications.
Five Ways to Kick off Your Journey
A structured approach for embarking on a Supply Chain AI journey is essential to a smooth transition. Companies need to define objectives, acquire the right tools and technology, prepare the data infrastructure, implement AI models, and continuously improve the system.
Here are five pivotal use cases for AI adoption that can be achieved with DemandAI+ and InventoryAI+ solutions. These scenarios not only showcase the potential impact of AI-first supply chain planning but also demonstrate versatile and far-reaching applications across various business aspects.
1. Demand sensing
Real-time visibility and insights into shorter-term demand enable improved service levels and higher forecast accuracy. This capability translates market-based demand information to allow supply chain organizations to detect short-term buying patterns. New mathematical techniques and near real-time demand signals can then be leveraged to improve supply chain response to unplanned demand changes – a transformational opportunity for any company.
2. Causal forecasting
Built-on causal forecasting isolates actual demand signals from market “noise.” By combining machine learning with Generative AI techniques, it can uncover complex patterns that are often missed, helping supply chain professionals focus on data that matters most to their business, customers, and overall growth.
3. New product introductions
Forecasting new product introductions with no sales history can be challenging, but artificial intelligence can be a valuable tool to help make these predictions more accurate. Supply chain systems can learn from evolving demand in real time to produce a more precise forecast with significantly less effort. Then, over time, downstream supply chain plans become more specific – leading to higher profitability, more-satisfied customers, and better synchronization across supply chain partners.
4. Inventory optimization
Applying artificial intelligence to inventory management provides immediate insights into inventory performance against the plan. This form of intelligent analytics opens the door to more-profitable inventory positions while giving supply chain planners real-time alerts and instant visibility into exceptions and proposed resolution of issues.
Better yet, with intelligent scoring, the intelligent scenario applies economic prioritization to focus more on the most-important opportunities – ultimately reducing bloated inventories while increasing service levels and avoiding deficits. Moreover, inventory planning capabilities can automate the identification of the best inventory policy for each SKU at every stocking location based on the most current information. Such data includes demand, demand variability, supply variability, lead time between facilities, inventory held at alternate stocking locations, and the types of stock at each facility.
Supply chain planners can also leverage an AI-automated inventory policies feature to determine which products are experiencing sporadic or “lumpy” demand, such as wide size ranges, spare parts, or industrial equipment. This approach allows supply chains to apply a stochastic replenishment planning tactic that can result in service-level improvements between 9% and 27% while lowering inventory levels and logistics costs.
5. Network optimization
The complexities in today’s supply chain network flows not only impede effective monitoring and management of goods movements but also increase a supply chain’s vulnerability to natural disasters and geopolitical tensions that can further compound delivery interruptions.
An innovative approach to network optimization can address this common issue by swiftly creating a digital twin of a supply chain. By employing Generative AI to explore potential future scenarios, this strategy enables supply chain planners to analyze and assess various configurations that can effectively manage costs, improve service, and align with emission reduction objectives. In addition, planners can simulate and evaluate diverse scenarios, allowing for proactive decision-making and strategic planning to navigate the complexities of the modern supply chain landscape.
A smooth path to true AI-first business transformation
By recognizing the unique and complementary relationship between machine learning and Generative AI, supply chain organizations have a one-of-a-kind opportunity to usher their companies into a new era of data intelligence. They can not only navigate the complexities of modern supply chains but also improve efficiencies, manage costs, enhance service levels, and create a more sustainable future for their supply chain and overall business.
Revolutionizing Supply Chain Planning with ChatGPT and AI-First Forecasting
Webinar: 31% of supply chain leaders are either developing proposals to begin or currently testing pilots for incorporate AI into their business. See why in this webinar
Watch for Free